Difficult sample mining method and system, terminal and storage medium
A sample and difficult technology, applied in terminals and storage media, difficult sample mining methods, and system fields, can solve problems such as different sampling probabilities, inapplicability, segmentation missed detection, etc., to alleviate the problem of category imbalance and improve model accuracy , to speed up the effect of model fitting
- Summary
- Abstract
- Description
- Claims
- Application Information
AI Technical Summary
Problems solved by technology
Method used
Image
Examples
Embodiment Construction
[0061] In order to make the purpose, technical solution and advantages of the present application clearer, the present application will be further described in detail below in conjunction with the accompanying drawings and embodiments. It should be understood that the specific embodiments described here are only used to explain the present application, not to limit the present application.
[0062] see figure 1 , is a flow chart of the difficult sample mining method in the embodiment of the present application. The difficult sample mining method of the embodiment of the present application includes the following steps:
[0063] S10: Collect medical image data with multiple organ labels, and randomly divide the medical image data into training set, verification set and test set;
[0064] In this step, the medical image data is abdominal CT data, specifically, it may also be medical image data of other parts.
[0065] S20: Construct a point cloud shape model including multipl...
PUM
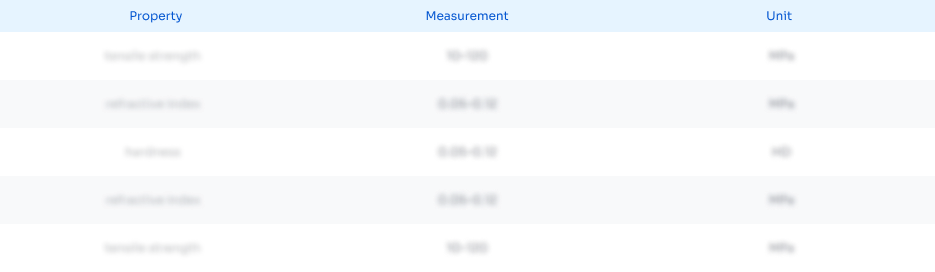
Abstract
Description
Claims
Application Information
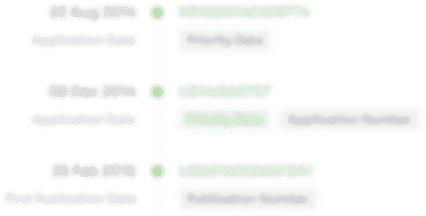
- Generate Ideas
- Intellectual Property
- Life Sciences
- Materials
- Tech Scout
- Unparalleled Data Quality
- Higher Quality Content
- 60% Fewer Hallucinations
Browse by: Latest US Patents, China's latest patents, Technical Efficacy Thesaurus, Application Domain, Technology Topic, Popular Technical Reports.
© 2025 PatSnap. All rights reserved.Legal|Privacy policy|Modern Slavery Act Transparency Statement|Sitemap|About US| Contact US: help@patsnap.com