Method for predicting permeability of porous medium based on LSTM (Long Short Term Memory)
A porous medium and prediction method technology, applied in the field of porous medium permeability prediction, can solve the problems of complex porous medium structure feature extraction, sample data compression, and difficult training, etc., and achieve the intuitive and clear feature extraction method, which is easy to implement Effect
- Summary
- Abstract
- Description
- Claims
- Application Information
AI Technical Summary
Problems solved by technology
Method used
Image
Examples
Embodiment
[0027] The effects of the present invention are verified below in conjunction with specific implementation examples.
[0028]In this example, 5000 semi-real three-dimensional porous media generated by Arash Rabbani were selected (Rabbani, Arash, et al. "DeePore: a deep learning workflow for rapid and comprehensive characterization of porous materials." Advances in Water Resources 146(2020): 103787.), each porous media volume is 256 3 voxel, with a voxel size of 5 μm. Permeability is calculated by a pore network model (PNM) and is measured in squares of pixels. Divide the porous medium into 256 binary images of 256×256 pixels according to the x-axis, where 1 value represents the solid phase skeleton and 0 value represents the pore structure. The porosity, ratio, and Euler number of each binary image can be obtained sequentially by python data processing and sklearn-image method, and after forming a one-dimensional feature sequence, it is collaged into a 256×3 feature sequence...
PUM
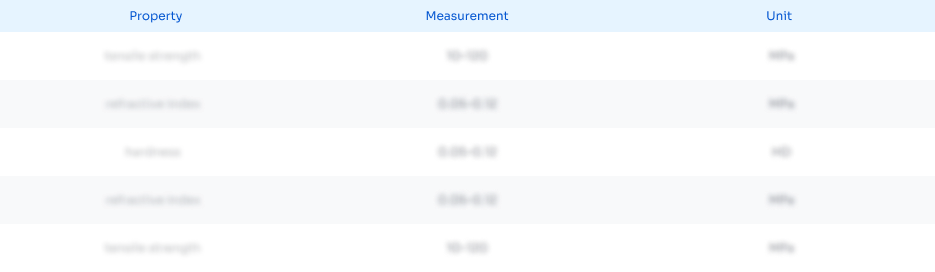
Abstract
Description
Claims
Application Information
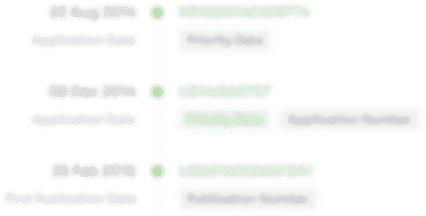
- R&D Engineer
- R&D Manager
- IP Professional
- Industry Leading Data Capabilities
- Powerful AI technology
- Patent DNA Extraction
Browse by: Latest US Patents, China's latest patents, Technical Efficacy Thesaurus, Application Domain, Technology Topic, Popular Technical Reports.
© 2024 PatSnap. All rights reserved.Legal|Privacy policy|Modern Slavery Act Transparency Statement|Sitemap|About US| Contact US: help@patsnap.com