Bearing residual life prediction method and system based on deep wavelet extreme learning machine
An extreme learning machine and life prediction technology, applied in the field of mechanical system condition monitoring and health management, can solve the problem of limited information provided by predictive maintenance, and achieve the effect of ensuring the accuracy of life prediction, reducing the amount of storage and processing data, and improving computing efficiency.
- Summary
- Abstract
- Description
- Claims
- Application Information
AI Technical Summary
Problems solved by technology
Method used
Image
Examples
Embodiment Construction
[0059] The following will clearly and completely describe the technical solutions in the embodiments of the present invention with reference to the accompanying drawings in the embodiments of the present invention. Obviously, the described embodiments are some of the embodiments of the present invention, but not all of them. Based on the embodiments of the present invention, all other embodiments obtained by persons of ordinary skill in the art without making creative efforts belong to the protection scope of the present invention.
[0060] In the description of the present invention, it should be understood that the terms "comprising" and "comprising" indicate the presence of described features, integers, steps, operations, elements and / or components, but do not exclude one or more other features, Presence or addition of wholes, steps, operations, elements, components and / or collections thereof.
[0061] It should also be understood that the terminology used in the descriptio...
PUM
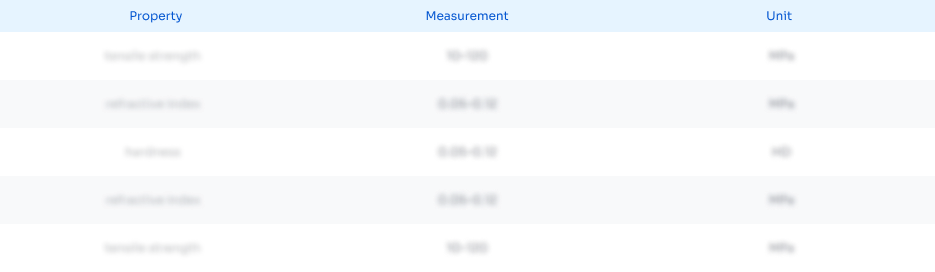
Abstract
Description
Claims
Application Information
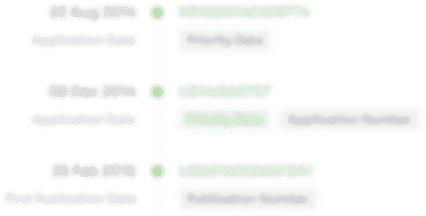
- R&D Engineer
- R&D Manager
- IP Professional
- Industry Leading Data Capabilities
- Powerful AI technology
- Patent DNA Extraction
Browse by: Latest US Patents, China's latest patents, Technical Efficacy Thesaurus, Application Domain, Technology Topic, Popular Technical Reports.
© 2024 PatSnap. All rights reserved.Legal|Privacy policy|Modern Slavery Act Transparency Statement|Sitemap|About US| Contact US: help@patsnap.com