Multi-scale fine-grained image recognition method and system based on multi-granularity attention
A technology of image recognition and attention, applied in the field of image processing, to achieve the effect of improving performance and classification accuracy
- Summary
- Abstract
- Description
- Claims
- Application Information
AI Technical Summary
Problems solved by technology
Method used
Image
Examples
Embodiment 1
[0053] combine Figure 1-Figure 5 As shown, this embodiment provides a fine-grained image recognition method based on a multi-scale neural network with multi-granularity attention, including the following steps:
[0054] Step 1. Multi-granularity feature extraction:
[0055] In this embodiment, ScaleNet is used as the feature extraction network, which includes N stages in total.
[0056] In this embodiment, the feature extraction network is divided into S stages through an attention-based multi-granularity structure, images of different granularity are input to different stages of the feature extraction network, and feature extraction is performed on images of different granularities in different stages to obtain different Raw feature maps for granularity.
[0057] Assuming the multi-grain structure based on attention k The input image for the stage is ,in
[0058] . here the first k The original feature map of the output of the stage is F k for:
[0059]
[00...
Embodiment 2
[0104] This embodiment provides a multi-scale fine-grained image recognition system based on multi-granularity attention, combining Figure 5 As shown in the network architecture diagram of the model used in the present invention, the fine-grained image recognition system includes an attention-based multi-granularity structure, a multi-granularity attention module, a parallel multi-scale convolution module, a feature fusion module, and a classifier. The multi-granularity structure based on attention divides the feature extraction network into several stages, and extracts the original feature maps of different granularity images in different stages; the multi-granularity attention module divides the original features of different granularities in each stage The image and its feature map with increased receptive field obtained through the convolution block are fused, and then for the fused feature map, attention weights are generated from the two domains of channel and space, and...
PUM
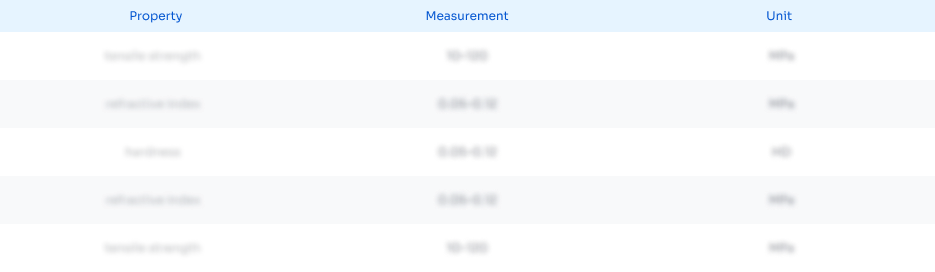
Abstract
Description
Claims
Application Information
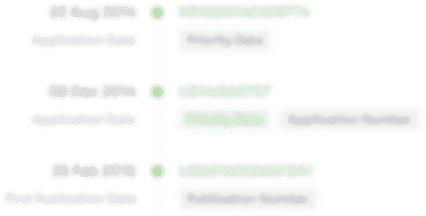
- R&D
- Intellectual Property
- Life Sciences
- Materials
- Tech Scout
- Unparalleled Data Quality
- Higher Quality Content
- 60% Fewer Hallucinations
Browse by: Latest US Patents, China's latest patents, Technical Efficacy Thesaurus, Application Domain, Technology Topic, Popular Technical Reports.
© 2025 PatSnap. All rights reserved.Legal|Privacy policy|Modern Slavery Act Transparency Statement|Sitemap|About US| Contact US: help@patsnap.com