Malicious domain name detection method based on comparative learning under limited training samples
A technology for training samples and domain name detection, which is applied in the field of cyberspace security, can solve problems such as imbalance, and achieve the effect of improving classification efficiency and information utilization
- Summary
- Abstract
- Description
- Claims
- Application Information
AI Technical Summary
Problems solved by technology
Method used
Image
Examples
Embodiment Construction
[0050] The technical solutions provided by the present invention will be described in detail below in conjunction with specific examples. It should be understood that the following specific embodiments are only used to illustrate the present invention and are not intended to limit the scope of the present invention.
[0051] The present invention proposes a malicious domain name detection method based on comparative learning under limited training samples, and the implementation steps are as follows: figure 1 As shown, it includes three parts. The first part is to train the feature extractor based on the Siamese network. The specific content is to first obtain the finite domain name training sample set in the form of label encoding, pair up the positive and negative sample pairs according to the attributes, and then design two BiLSTM-based sub-networks with the same structure and different weights, and then use the twin network framework to connect the two sub-networks to desi...
PUM
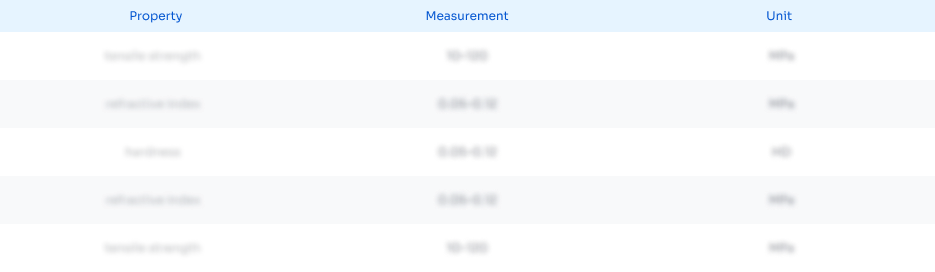
Abstract
Description
Claims
Application Information
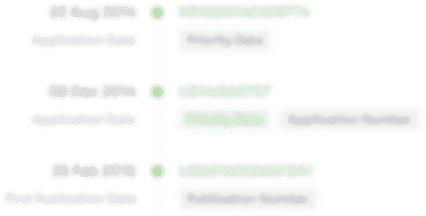
- Generate Ideas
- Intellectual Property
- Life Sciences
- Materials
- Tech Scout
- Unparalleled Data Quality
- Higher Quality Content
- 60% Fewer Hallucinations
Browse by: Latest US Patents, China's latest patents, Technical Efficacy Thesaurus, Application Domain, Technology Topic, Popular Technical Reports.
© 2025 PatSnap. All rights reserved.Legal|Privacy policy|Modern Slavery Act Transparency Statement|Sitemap|About US| Contact US: help@patsnap.com