Smart power grid partition network reconstruction method based on multi-agent reinforcement learning
A reinforcement learning, multi-agent technology, applied in the direction of AC network circuits, electrical components, circuit devices, etc., can solve the problems of slow processing speed, lack of countermeasures for risks, and huge calculation amount of optimization algorithms, so as to achieve good response measures and training. The effect of high efficiency and fast decision-making
- Summary
- Abstract
- Description
- Claims
- Application Information
AI Technical Summary
Problems solved by technology
Method used
Image
Examples
Embodiment Construction
[0041] The present invention will be further described in detail below in conjunction with the accompanying drawings and specific embodiments.
[0042] An automatic decision-making method for smart grid partitions based on multi-agent reinforcement learning, its overall flow chart refers to figure 1 , the method includes the following steps:
[0043] Step 1: Divide the power grid into N regions according to the needs of power grid operation, and construct the basic elements of multi-agent reinforcement learning (MARL), including environment, agent, state, observation, action, and reward function.
[0044] Step 2: Run the power system simulation environment to create the initial operating state data set of the power system.
[0045] Step 3: Construct a deep neural network model, and train the decision-making agent with enhanced inter-agent learning (RIAL).
[0046] Step 4: Use the trained agent to provide strategies for grid control.
[0047] The present invention also inclu...
PUM
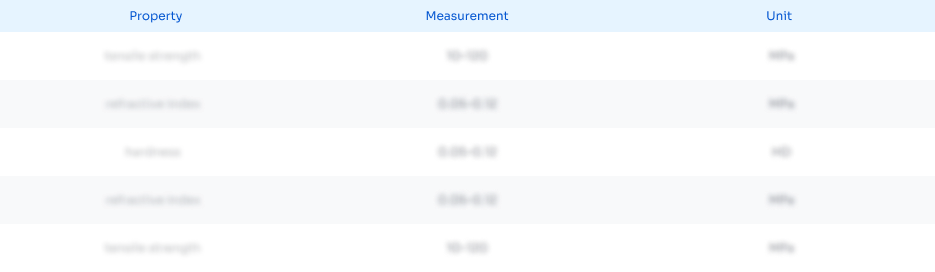
Abstract
Description
Claims
Application Information
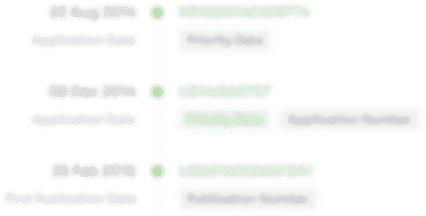
- R&D
- Intellectual Property
- Life Sciences
- Materials
- Tech Scout
- Unparalleled Data Quality
- Higher Quality Content
- 60% Fewer Hallucinations
Browse by: Latest US Patents, China's latest patents, Technical Efficacy Thesaurus, Application Domain, Technology Topic, Popular Technical Reports.
© 2025 PatSnap. All rights reserved.Legal|Privacy policy|Modern Slavery Act Transparency Statement|Sitemap|About US| Contact US: help@patsnap.com