Image super-division method based on progressive residual generative adversarial network
A network and residual technology, applied in the field of image processing, can solve problems such as slowing down network processing efficiency, complex network structure, and inability to make full use of input feature information, and achieve the effect of improving super-resolution effect, low cost, and easy implementation
- Summary
- Abstract
- Description
- Claims
- Application Information
AI Technical Summary
Problems solved by technology
Method used
Image
Examples
Embodiment Construction
[0040] The method of the present invention will be further described below in conjunction with the accompanying drawings and embodiments.
[0041] An image super-resolution method based on progressive residual generation confrontation network, the steps are as follows:
[0042] Step 1: Perform low-resolution sampling preprocessing on the image. For a given natural real image I r , and its dimension is M*N*C, where M and N represent the width and height of the image respectively, M=N=256; C=3 represents the dimension of the image. The image is continuously down-sampled according to the down-sampling parameter α until the size of the image is about 1 / 10 of the original image. This article uses a 13-layer subnetwork (GAN j , 0≤j≤12, the larger the network layer, the lower the image resolution of j) constitutes the overall generative confrontation network, the ultimate goal is to build a reconstructed image that generates 4 times the super-resolution of the original image, and ...
PUM
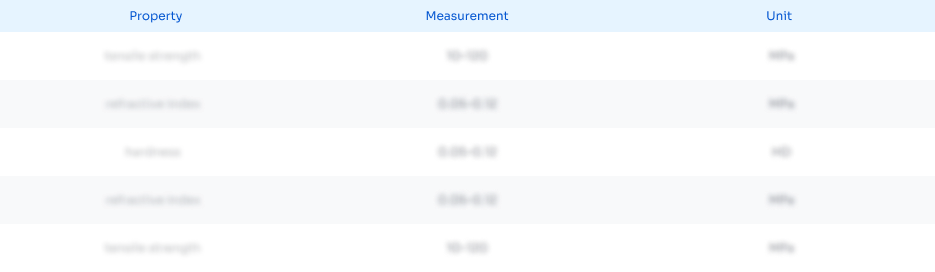
Abstract
Description
Claims
Application Information
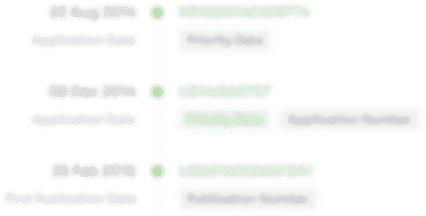
- R&D Engineer
- R&D Manager
- IP Professional
- Industry Leading Data Capabilities
- Powerful AI technology
- Patent DNA Extraction
Browse by: Latest US Patents, China's latest patents, Technical Efficacy Thesaurus, Application Domain, Technology Topic, Popular Technical Reports.
© 2024 PatSnap. All rights reserved.Legal|Privacy policy|Modern Slavery Act Transparency Statement|Sitemap|About US| Contact US: help@patsnap.com