Bearing fault diagnosis method based on dimension transformation convolution depth forest
A fault diagnosis and forest technology, applied in neural learning methods, testing of mechanical components, pattern recognition in signals, etc., can solve problems such as large diagnostic errors, difficulty in information extraction, good and bad prediction results, etc., and achieve high accuracy High rate and accuracy, good robustness
- Summary
- Abstract
- Description
- Claims
- Application Information
AI Technical Summary
Problems solved by technology
Method used
Image
Examples
Embodiment Construction
[0033] The present invention will be further described below in conjunction with the accompanying drawings:
[0034]The present invention is mainly aimed at deep forest multi-granularity scanning that will generate a large number of subset samples, occupy a large amount of space, easily generate data redundancy and can only run on the CPU, and the running speed is indeed much lower than running on the GPU. CNN has a large number of hyperparameters and has a powerful representation learning ability. But the SoftMax function used for classification in the last layer is somewhat simple. Therefore, the multi-granularity scanning in the deep forest is replaced by CNN, and the cascade forest is used for classification, and CNN can be run using GPU. The present invention can achieve higher accuracy rate and lower time consumption than single CNN and deep forest. CNN structure such as image 3 As shown, the convolutional deep forest architecture is as Figure 4 As shown, the whole...
PUM
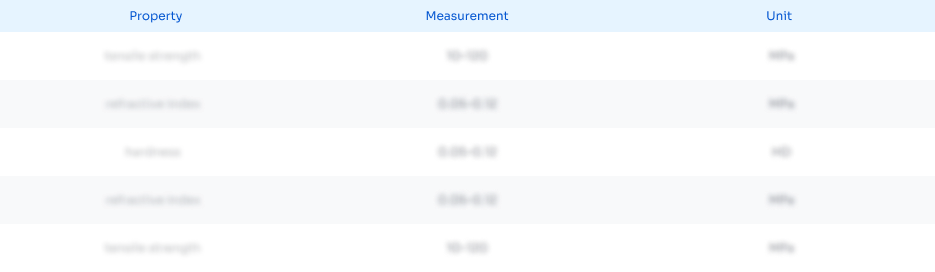
Abstract
Description
Claims
Application Information
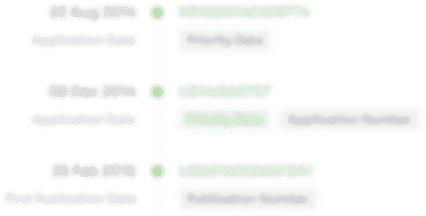
- R&D Engineer
- R&D Manager
- IP Professional
- Industry Leading Data Capabilities
- Powerful AI technology
- Patent DNA Extraction
Browse by: Latest US Patents, China's latest patents, Technical Efficacy Thesaurus, Application Domain, Technology Topic.
© 2024 PatSnap. All rights reserved.Legal|Privacy policy|Modern Slavery Act Transparency Statement|Sitemap