Adaptive medical image classification method and system based on Transform
A technology for medical imaging and classification methods, applied in biological neural network models, instruments, character and pattern recognition, etc., can solve the problems of increased network calculation, less related information, and unfavorable feature capture, and achieves the goal of reducing model redundancy. Effect
- Summary
- Abstract
- Description
- Claims
- Application Information
AI Technical Summary
Problems solved by technology
Method used
Image
Examples
Embodiment Construction
[0032] The present invention proposes an adaptive medical imaging classification method based on TRANSFORMER, including the following steps:
[0033] S1, first, obtain the original image (image), zoom the original image to the unified size to transfer to the convolutional neural network, by convolutional neural network acquisition base information.
[0034] S2, second, the basic feature drawn obtained in S1 is transmitted to the channel attention network structure to obtain a passage attention after weighted.
[0035] S3, again, pass the channel attention feature map to the Transformer network to obtain the feature vectors after the Transformer process.
[0036] S4, finally the feature vector obtained by S3 is input to the full connection layer, and the full connection layer direct outputs the final classification result.
[0037] In order to make the above features and effects of the present invention, it is more clearly understood, and the following embodiments are apparent, and...
PUM
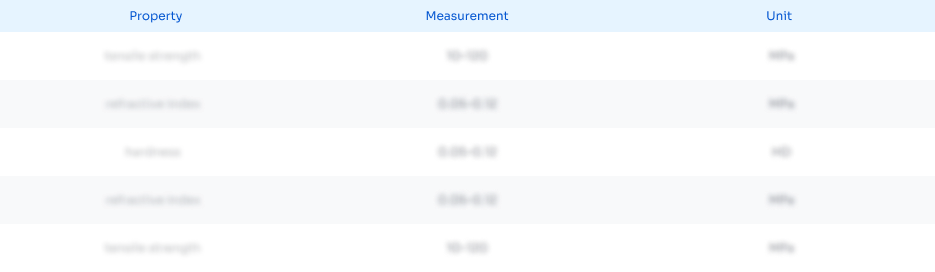
Abstract
Description
Claims
Application Information
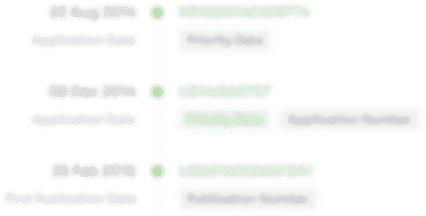
- R&D Engineer
- R&D Manager
- IP Professional
- Industry Leading Data Capabilities
- Powerful AI technology
- Patent DNA Extraction
Browse by: Latest US Patents, China's latest patents, Technical Efficacy Thesaurus, Application Domain, Technology Topic, Popular Technical Reports.
© 2024 PatSnap. All rights reserved.Legal|Privacy policy|Modern Slavery Act Transparency Statement|Sitemap|About US| Contact US: help@patsnap.com