Short-term wind power generation prediction method, device, equipment and storage medium
A prediction method and power generation technology, applied in the field of new energy power generation prediction, can solve problems such as uneven results, and achieve the effect of improving prediction accuracy, generalization ability and stability
- Summary
- Abstract
- Description
- Claims
- Application Information
AI Technical Summary
Problems solved by technology
Method used
Image
Examples
Embodiment 1
[0047] Such as figure 1 As shown, a short-term wind power forecasting method includes the following steps:
[0048]S110. Obtain historical meteorological feature data and corresponding historical power generation, and use the Pearson correlation coefficient method to calculate the correlation coefficient between meteorological features and power generation;
[0049] S120. Determine the main meteorological features that affect the power generation according to the correlation coefficient, and combine the historical data of the main meteorological features with the historical data of the power generation to obtain a target data set;
[0050] S130, setting and initializing the parameters of the multiverse algorithm and the nuclear extreme learning machine;
[0051] S140. Divide the target data set into a training set and a test set, and use the training set to train a kernel extreme learning machine;
[0052] S150. Run the multiverse algorithm to obtain the optimal combination ...
Embodiment 2
[0062] Such as figure 2 As shown, a short-term wind power forecasting method, including:
[0063] S210. Obtain historical meteorological feature data and corresponding historical power generation power, and use the Pearson correlation coefficient method to calculate the correlation coefficient between meteorological features and power generation power;
[0064] S220. Determine the main meteorological features affecting the power generation according to the correlation coefficient, and combine the historical data of the main meteorological features with the historical data of the power generation to obtain a target data set;
[0065] S230, setting the development accuracy of the multiverse algorithm, the maximum number of iterations, the population dimension, population size and population number in the population, and setting the kernel function type of the kernel extreme learning machine;
[0066] S240, run the whale optimization algorithm multiple times, save the optimal p...
Embodiment 3
[0076] Such as image 3 As shown, a short-term wind power forecasting method, including:
[0077] S310. Obtain historical meteorological feature data and corresponding historical power generation power, and use the Pearson correlation coefficient method to calculate the correlation coefficient between meteorological features and power generation power;
[0078] S320. Determine the main meteorological features that affect the power generation according to the correlation coefficient, and combine the historical data of the main meteorological features with the historical data of the power generation to obtain a target data set;
[0079] S330, setting and initializing the parameters of the multiverse algorithm and the nuclear extreme learning machine;
[0080] S340. Divide the target data set into a training set and a test set, and use the training set to train a kernel extreme learning machine;
[0081] S350. Calculate the initial universe individual fitness value of the multi...
PUM
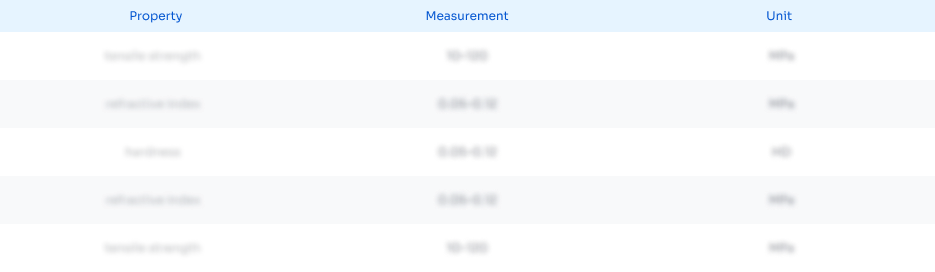
Abstract
Description
Claims
Application Information
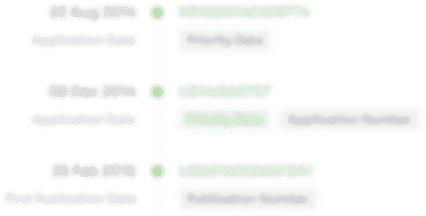
- R&D Engineer
- R&D Manager
- IP Professional
- Industry Leading Data Capabilities
- Powerful AI technology
- Patent DNA Extraction
Browse by: Latest US Patents, China's latest patents, Technical Efficacy Thesaurus, Application Domain, Technology Topic, Popular Technical Reports.
© 2024 PatSnap. All rights reserved.Legal|Privacy policy|Modern Slavery Act Transparency Statement|Sitemap|About US| Contact US: help@patsnap.com