Wind power prediction method based on AFSA-GNN
A technology of AFSA-GNN and wind power prediction, applied in the field of wind power, can solve the problems of reducing model speed and accuracy, slow convergence speed, influence, etc., and achieve the effect of improving optimization speed and prediction accuracy
- Summary
- Abstract
- Description
- Claims
- Application Information
AI Technical Summary
Problems solved by technology
Method used
Image
Examples
Embodiment Construction
[0039] In order to make the structure and advantages of the present application clearer, the structure of the present application will be further described below in conjunction with the accompanying drawings.
[0040] Specifically, the wind power prediction method based on AFSA-GNN proposed in the embodiment of this application, such as figure 1 shown, including:
[0041] S1, cleaning and normalizing the obtained wind power data;
[0042] S2, build the RNN model, and determine the number of hidden layer nodes of the RNN model;
[0043] S3, performing a regression operation based on the obtained RNN model to obtain the predicted power, and constructing an objective function combining the minimum root mean square error of the predicted power and the measured power;
[0044] S4, initialize various parameters of the AFSA algorithm, and use the steps of the AFSA algorithm to optimize the weight of the RNN model;
[0045] S5, inputting the wind power data processed in step S1 int...
PUM
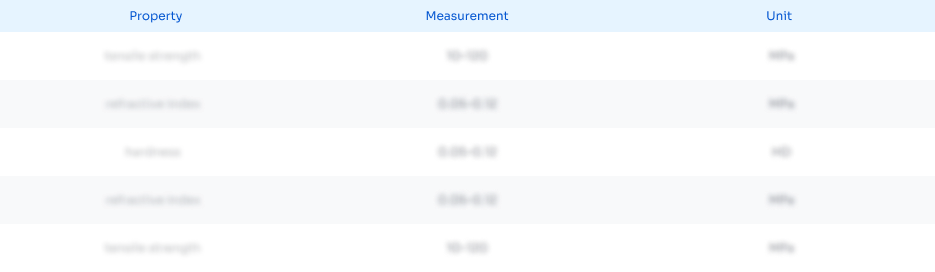
Abstract
Description
Claims
Application Information
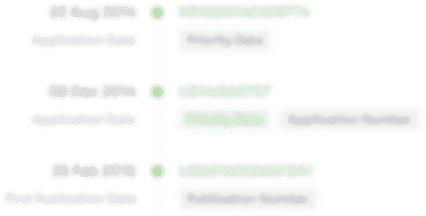
- R&D Engineer
- R&D Manager
- IP Professional
- Industry Leading Data Capabilities
- Powerful AI technology
- Patent DNA Extraction
Browse by: Latest US Patents, China's latest patents, Technical Efficacy Thesaurus, Application Domain, Technology Topic.
© 2024 PatSnap. All rights reserved.Legal|Privacy policy|Modern Slavery Act Transparency Statement|Sitemap