Service elastic expansion and contraction method based on emergency detection
An emergency and elastic technology, applied in the field of cloud computing, can solve problems such as inaccurate elastic scaling strategy, error in forecast results, and slow response speed, so as to improve scheduling accuracy, maintain scheduling stability, and reduce operation and maintenance costs Effect
- Summary
- Abstract
- Description
- Claims
- Application Information
AI Technical Summary
Problems solved by technology
Method used
Image
Examples
Embodiment 1
[0061] like figure 1 As shown, a service elastic scaling method based on emergency detection, the method includes the following steps:
[0062] S1: Start with the status of the obtained service as a work cycle;
[0063] S2: Obtain the previous workload data of the service, and input the trained long-term prediction model for multi-step probability prediction to obtain prediction results at multiple future time points. The prediction results include the prediction results at each time point, the possible range of workloads;
[0064] S3: Calculate the multi-step deviation vector according to the prediction results of multiple future time points;
[0065] S4: and update the upper bound value of the confidence interval of the multi-step probability prediction result according to the prediction results of multiple future time points;
[0066] S5: if there are at least two values in the multi-step deviation vector that are both greater than the preset threshold, it is considere...
Embodiment 2
[0084] Based on the service elastic scaling method described in Embodiment 1, this embodiment further provides a service elastic scaling system based on emergency detection, such as figure 2 , image 3 As shown, the system includes a monitoring component C100, a workload prediction component C110, an emergency situation judgment component C120, and an elastic scaling component C130; wherein the workload prediction component C110 includes a long-term probability prediction module C111, a long-term probability prediction module C112, a trend prediction module C113; the elastic scaling component C130 includes a non-emergency scaler C131 and an emergency scaler C132.
[0085] Starting with the state of the service obtained by the monitoring component C100 as the work cycle, at this time, the monitoring component C100 will store the index data representing the current state of the service in the external time series database, wherein the index data includes the average of the serv...
Embodiment 3
[0107] For the prediction model involved in the workload prediction component C110, the machine learning and neural network training methods commonly used in the industry can be used, and details are not described here.
[0108] In the non-burst scaler C131, the near-end policy optimization model in the non-burst scaler C131 is trained using the following reward function to ensure that the method can work properly.
[0109]
[0110]
[0111] r=a×vc+(1-a)×vr
[0112] Among them, vc represents the reward function component related to the CPU, vr represents the reward function component related to the average response time, r is the reward function value calculated according to the current CPU occupancy rate and response time, and a is a hyperparameter used to adjust The weight of CPU and response time is generally 0.5; cpu is the average CPU usage of the service instance, and the value ranges from 0 to 100. res is the average response time of the service instance, in mill...
PUM
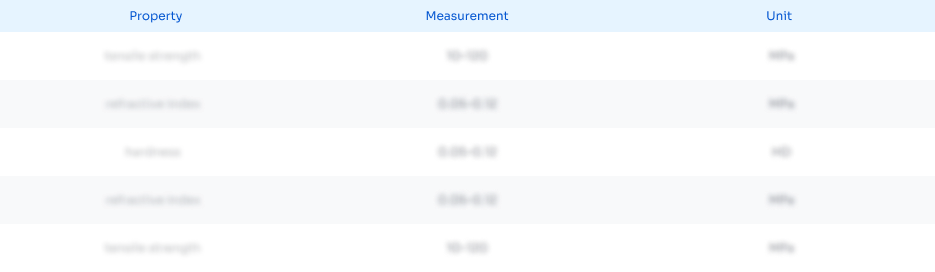
Abstract
Description
Claims
Application Information
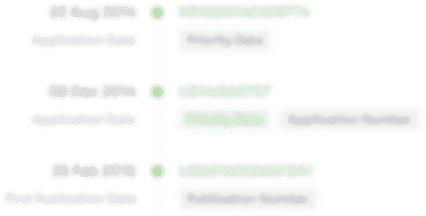
- Generate Ideas
- Intellectual Property
- Life Sciences
- Materials
- Tech Scout
- Unparalleled Data Quality
- Higher Quality Content
- 60% Fewer Hallucinations
Browse by: Latest US Patents, China's latest patents, Technical Efficacy Thesaurus, Application Domain, Technology Topic, Popular Technical Reports.
© 2025 PatSnap. All rights reserved.Legal|Privacy policy|Modern Slavery Act Transparency Statement|Sitemap|About US| Contact US: help@patsnap.com