Remaining oil distribution prediction method based on autoregressive network model
A technology of network model and distribution prediction, applied in the direction of biological neural network model, neural learning method, CAD numerical modeling, etc., can solve the problem of large amount of calculation, dynamic parameters that cannot be used to predict the distribution of remaining oil in the reservoir, long time consumption, etc. problem, to achieve the effect of saving time, improving prediction accuracy and accuracy, and promoting the value of application
- Summary
- Abstract
- Description
- Claims
- Application Information
AI Technical Summary
Problems solved by technology
Method used
Image
Examples
Embodiment
[0086] In order to prove the feasibility of the method of the present invention, a verification experiment was carried out by collecting real data of an oilfield block.
[0087] There are 9 wells in this oilfield block, including 4 water injection wells and 5 production wells, and the well location layout adopts the reverse five-point method model. In this experiment, constant pressure mining was adopted, and the bottomhole flow pressure was fixed. The size of the permeability field is 80×80, and the mean and variance of the permeability are 5.3 and 0.8, respectively. A total of 600 samples were generated in this experiment, of which 400 samples were used for training and 200 samples were used for testing.
[0088] Based on above-mentioned data, adopt the method of the present invention to carry out the concrete steps of residual oil distribution prediction as:
[0089] Step 1. Determine the influencing factors of remaining oil distribution. Starting from the basic seepage d...
PUM
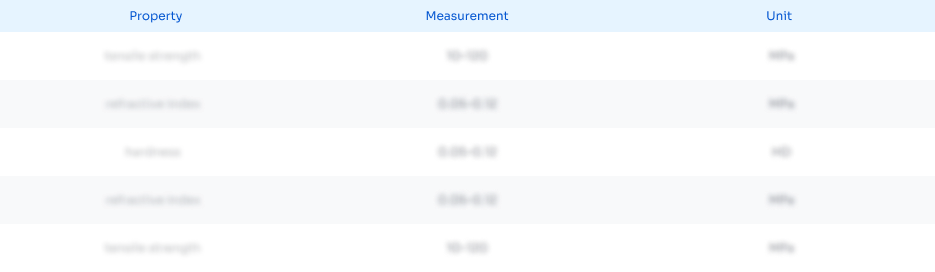
Abstract
Description
Claims
Application Information
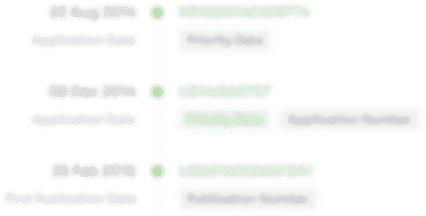
- R&D
- Intellectual Property
- Life Sciences
- Materials
- Tech Scout
- Unparalleled Data Quality
- Higher Quality Content
- 60% Fewer Hallucinations
Browse by: Latest US Patents, China's latest patents, Technical Efficacy Thesaurus, Application Domain, Technology Topic, Popular Technical Reports.
© 2025 PatSnap. All rights reserved.Legal|Privacy policy|Modern Slavery Act Transparency Statement|Sitemap|About US| Contact US: help@patsnap.com