Rotary machinery fault diagnosis method based on zero trial learning and feature extraction
A technology of feature extraction and rotating machinery, which is applied to computer components, character and pattern recognition, instruments, etc., can solve the problems of many monitoring points, scarcity of fault data, and long monitoring cycle, so as to prevent potential dangers and solve cross-data The effect of set deviation and high prediction accuracy
- Summary
- Abstract
- Description
- Claims
- Application Information
AI Technical Summary
Problems solved by technology
Method used
Image
Examples
Embodiment Construction
[0037] Below in conjunction with embodiment the present invention is described in further detail:
[0038] A fault diagnosis method for rotating machinery based on zero-trial learning and feature extraction, including the following steps:
[0039] S1. Acquire the data sets of bearings and gears required for the experiment, and divide the corresponding data sets into training set, verification set and test set.
[0040] S2. Data preprocessing and feature extraction: To normalize the data, it is first necessary to determine the length of a single sample. After experimental comparison, it is found that when the one-dimensional data is cut into a length of 2048, the effect is better. Therefore, in the preprocessing process, the data samples are first cut into sequences with a length of 2048. In the process of feature processing of data samples, the WDCNN network is used for feature extraction. The network structure has 5 convolutional layers, and finally the output of the fully c...
PUM
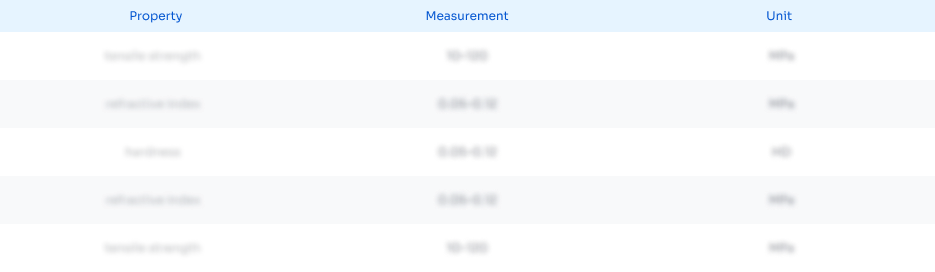
Abstract
Description
Claims
Application Information
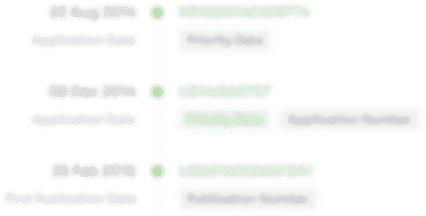
- R&D Engineer
- R&D Manager
- IP Professional
- Industry Leading Data Capabilities
- Powerful AI technology
- Patent DNA Extraction
Browse by: Latest US Patents, China's latest patents, Technical Efficacy Thesaurus, Application Domain, Technology Topic, Popular Technical Reports.
© 2024 PatSnap. All rights reserved.Legal|Privacy policy|Modern Slavery Act Transparency Statement|Sitemap|About US| Contact US: help@patsnap.com