Estimation and modeling method for charging load of electric vehicle
A technology for electric vehicles and charging loads, applied in neural learning methods, load forecasting and calculations in AC networks, etc., can solve problems such as low accuracy of model evaluation, and achieve the goal of saving travel time, high model accuracy, and scientific modeling methods Effect
- Summary
- Abstract
- Description
- Claims
- Application Information
AI Technical Summary
Problems solved by technology
Method used
Image
Examples
Embodiment Construction
[0029] The present invention is further described in detail by specific embodiments.
[0030] (1) Obtain the travel trajectory of electric vehicles, and explore the travel modes and charging modes of electric vehicle users
[0031]Through the GPS point of the electric vehicle to obtain the electric vehicle travel time, travel location, driving time, residence time and residence location (the acquisition of the user's travel mode), combined with the overlap between the parking position and the charging station, to determine whether the user has charging behavior and the battery charge state (SOC) when the electric vehicle is charged, to obtain the user's charging mode, the charging mode includes driving characteristics, charging mode, driving behavior and charging behavior. At the same time, due to the complex law of battery change of electric vehicles, the degree of non-linearity of data, it is difficult to establish an accurate mathematical model to describe it, the method by the...
PUM
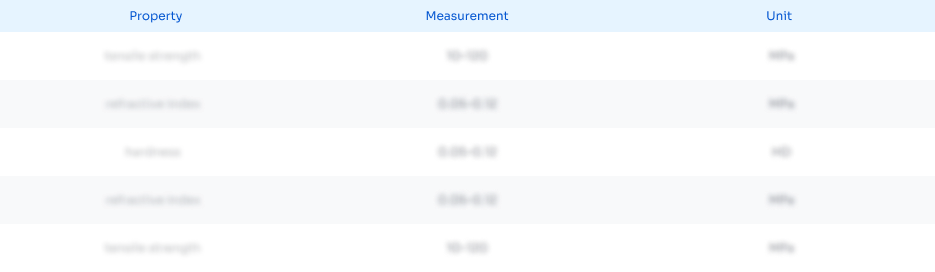
Abstract
Description
Claims
Application Information
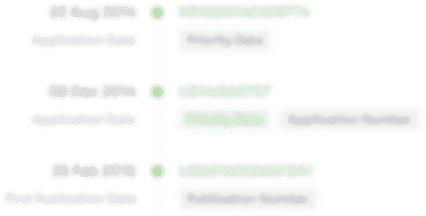
- R&D
- Intellectual Property
- Life Sciences
- Materials
- Tech Scout
- Unparalleled Data Quality
- Higher Quality Content
- 60% Fewer Hallucinations
Browse by: Latest US Patents, China's latest patents, Technical Efficacy Thesaurus, Application Domain, Technology Topic, Popular Technical Reports.
© 2025 PatSnap. All rights reserved.Legal|Privacy policy|Modern Slavery Act Transparency Statement|Sitemap|About US| Contact US: help@patsnap.com