RGB-D image semantic segmentation method based on multi-modal feature fusion
A RGB-D, feature fusion technology, applied in the field of computer vision, to achieve the effect of good complexity and diversity
- Summary
- Abstract
- Description
- Claims
- Application Information
AI Technical Summary
Problems solved by technology
Method used
Image
Examples
Embodiment 1
[0043] A RGB-D image semantic segmentation method based on multimodal feature fusion, comprising the following steps:
[0044] Step 1, data preprocessing, convert a single-channel depth image into a three-channel HHA image, and the three channels represent the height of the horizontal parallax above the ground, the local surface normal of the pixel, and the inferred angle of the direction of gravity;
[0045] Step 2, take the RGB and HHA images as input data, and input the attention-guided multi-modal cross-fusion segmentation network model (such as figure 1 shown), the model follows an encoder-decoder structure, where the encoder extracts semantic features from the input, and the decoder recovers the input resolution using upsampling techniques, assigning a semantic class to each input pixel.
[0046] The encoder uses asymmetric dual-stream branches for RGB and HHA images, including RGB encoder and depth encoder, which use ResNet-101 network and ResNet-50 network as backbone ...
Embodiment 2
[0069] The segmentation network model proposed by the present invention is applied on the public RGB-D indoor dataset NYUD V2, and experiments show the effectiveness of the network model.
[0070] The NYUD V2 dataset contains 1449 labeled RGB-D image pairs from 464 different indoor scenes in 3 cities, of which 796 image pairs are used for training and 654 image pairs are used for testing. The way objects are classified into 40 categories. The effectiveness of the proposed segmentation network ACFNet is shown by providing segmentation visualization comparison results. The network structure after removing the feature fusion module ACFM and the global-local feature extraction module (GL) is recorded as the baseline network (Baseline). Figure 5 Partial visualization results of ACFNet on the public dataset NYUD V2 are shown in , where the first, second, and third columns represent RGB images, HHA images, and semantic labels in turn, and the fourth and fifth columns represent the ...
PUM
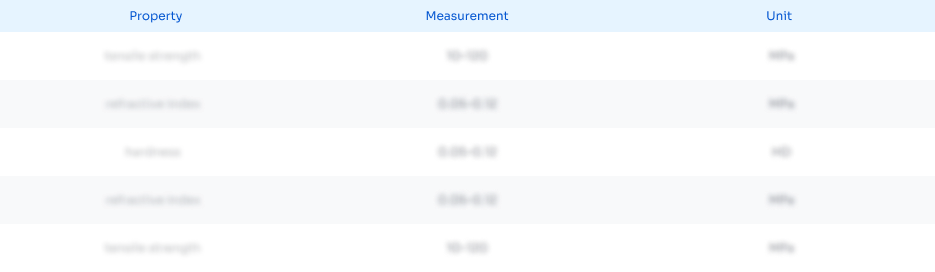
Abstract
Description
Claims
Application Information
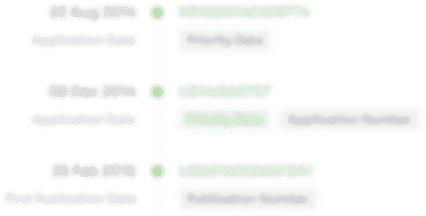
- R&D
- Intellectual Property
- Life Sciences
- Materials
- Tech Scout
- Unparalleled Data Quality
- Higher Quality Content
- 60% Fewer Hallucinations
Browse by: Latest US Patents, China's latest patents, Technical Efficacy Thesaurus, Application Domain, Technology Topic, Popular Technical Reports.
© 2025 PatSnap. All rights reserved.Legal|Privacy policy|Modern Slavery Act Transparency Statement|Sitemap|About US| Contact US: help@patsnap.com