Adversarial sample generation method based on target detection model feature vector migration
A technology of adversarial samples and target detection, applied in the field of computer vision and deep learning, can solve the problems of adversarial sample distortion, concealment deterioration, increase of anti-noise, etc., and achieve strong attack effect and good transferability
- Summary
- Abstract
- Description
- Claims
- Application Information
AI Technical Summary
Problems solved by technology
Method used
Image
Examples
Embodiment Construction
[0061] This embodiment proposes an adversarial sample generation method based on feature vector migration of a target detection model. The adversarial sample generation method includes three types of entities: several image samples, several deep learning models and an attacker. The image samples are several pictures taken in real scenes, such as: portraits, animals, urban traffic, etc. In this embodiment, the target detection model is used as a representative of the deep learning model. The target detection model is a deep learning model based on image tasks, the model can classify and locate the target object on the image sample, and output the detection result.
[0062] like figure 1 As shown, the target detection model can distinguish the foreground of a given image, that is, the target object and the background part, and identify the category of the foreground target object on the image, and select the corresponding position of each target object, that is, the detection f...
PUM
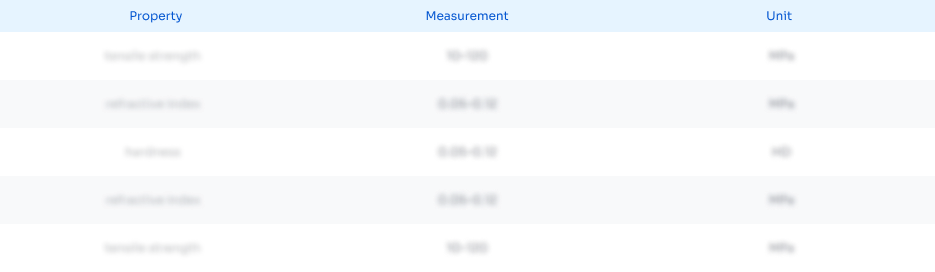
Abstract
Description
Claims
Application Information
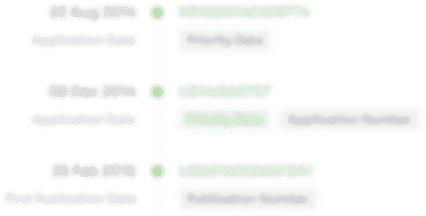
- R&D
- Intellectual Property
- Life Sciences
- Materials
- Tech Scout
- Unparalleled Data Quality
- Higher Quality Content
- 60% Fewer Hallucinations
Browse by: Latest US Patents, China's latest patents, Technical Efficacy Thesaurus, Application Domain, Technology Topic, Popular Technical Reports.
© 2025 PatSnap. All rights reserved.Legal|Privacy policy|Modern Slavery Act Transparency Statement|Sitemap|About US| Contact US: help@patsnap.com