Federal learning-based sequence recommendation method and system
A recommendation method and federated technology, applied in integrated learning, instrumentation, computing, etc., can solve the problems of ignoring the personalization of the client-side model, affecting the performance of the global model, and extreme performance of the local model, so as to improve the personalized experience and avoid Homogenization problem, the effect of a wide range of applications
- Summary
- Abstract
- Description
- Claims
- Application Information
AI Technical Summary
Problems solved by technology
Method used
Image
Examples
Embodiment Construction
[0024] The present invention will be described in detail below with reference to the accompanying drawings and specific embodiments.
[0025] The federated learning architecture proposed by the present invention is as follows: figure 1 As shown in the figure, serialization modeling is performed using reinforcement learning under the condition that user data and information are not available locally. During the process of communication between the local and the central server, the transmitted content is no longer the original data, but the model parameters of a certain proportion of the client. It solves the problem of data privacy protection and the low efficiency of centralized sampling, reduces the economic loss caused by cold start, and is of great significance for large-scale recommendation scenarios.
[0026] like figure 2 As shown, the sequence recommendation method based on federated learning mainly includes the following steps:
[0027] Step 1: The central server pr...
PUM
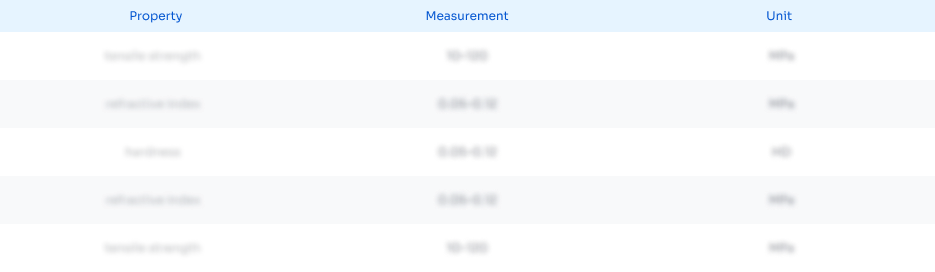
Abstract
Description
Claims
Application Information
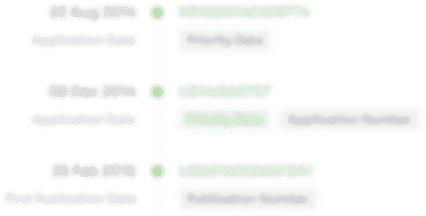
- R&D
- Intellectual Property
- Life Sciences
- Materials
- Tech Scout
- Unparalleled Data Quality
- Higher Quality Content
- 60% Fewer Hallucinations
Browse by: Latest US Patents, China's latest patents, Technical Efficacy Thesaurus, Application Domain, Technology Topic, Popular Technical Reports.
© 2025 PatSnap. All rights reserved.Legal|Privacy policy|Modern Slavery Act Transparency Statement|Sitemap|About US| Contact US: help@patsnap.com