Improved few-sample target detection method based on Faster RCNN
A target detection and sample technology, applied in the field of deep learning target detection and few-sample learning, can solve the problems of restricting the application and promotion of target detection methods, covering a single task, and a single application scenario, so as to improve detection performance, improve detection accuracy, The effect of reducing within-class variance
- Summary
- Abstract
- Description
- Claims
- Application Information
AI Technical Summary
Problems solved by technology
Method used
Image
Examples
Embodiment Construction
[0027] The specific embodiments of the present invention will be described in detail below with reference to the accompanying drawings. But the following examples are only limited to explain the present invention, and the protection scope of the present invention should include the full content of the claims; and through the following examples, those skilled in the art can realize the full content of the claims of the present invention.
[0028] In this specific embodiment, the method for detecting a few-sample target mainly includes the following steps:
[0029] Step 1: Input Image
[0030] The input images are divided into support set images and query set images. The image to be detected is the query set image, which contains unlabeled target samples, and the support set image is a small number of images containing labeled target samples.
[0031] Step 2: Feature Extraction
[0032] The support set image and the query image to be detected are regarded as the support image ...
PUM
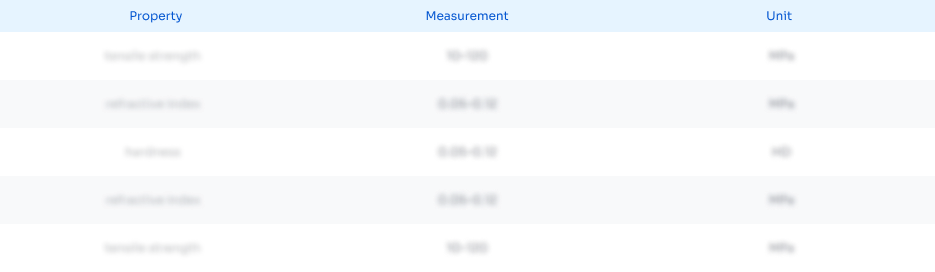
Abstract
Description
Claims
Application Information
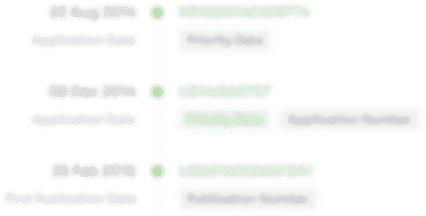
- R&D Engineer
- R&D Manager
- IP Professional
- Industry Leading Data Capabilities
- Powerful AI technology
- Patent DNA Extraction
Browse by: Latest US Patents, China's latest patents, Technical Efficacy Thesaurus, Application Domain, Technology Topic, Popular Technical Reports.
© 2024 PatSnap. All rights reserved.Legal|Privacy policy|Modern Slavery Act Transparency Statement|Sitemap|About US| Contact US: help@patsnap.com