Hyperspectral image change detection method based on spatio-temporal joint graph attention mechanism
An attention mechanism and change detection technology, which is applied in the field of remote sensing image processing, can solve the problems of feature extraction limitation, inability to adapt to topology structure, and inability to effectively use land cover correlation, etc., to improve the accuracy of change detection and reduce dependence.
- Summary
- Abstract
- Description
- Claims
- Application Information
AI Technical Summary
Problems solved by technology
Method used
Image
Examples
Embodiment Construction
[0108] The technical solution of the present invention is: a hyperspectral image change detection method based on a spatiotemporal joint graph attention mechanism, such as figure 1 Follow the steps below in sequence:
[0109] Step 1. Separate hyperspectral images of T1 time-phase X 1 ∈R H×W×C , T2-phase hyperspectral image X 2 ∈R H×W×C and the corresponding label graph g∈R H×W preprocessing
[0110] Step 1.1. Convert the input T1-phase hyperspectral image to X 1 ∈R H×W×C Hyperspectral image X with T2 phase 2 ∈R H×W×C Connect according to the channel dimension to obtain the T3 time-phase hyperspectral image X 3 ∈R H×W×2C , where H×W is the spatial size of the image, and C is the spectral length;
[0111] Step 1.2. Map the hyperspectral images of T1 and T2 phases to the two-dimensional space R HW×C , mapping the hyperspectral image of T3 phase to the two-dimensional space R HW×2C , using the packaged data standardization method sklearn.preprocessing.StandScaler to s...
PUM
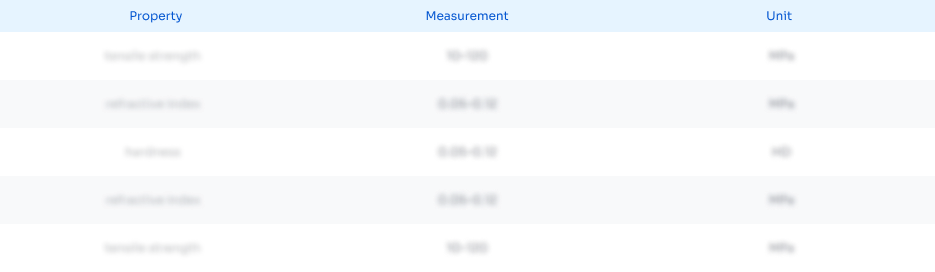
Abstract
Description
Claims
Application Information
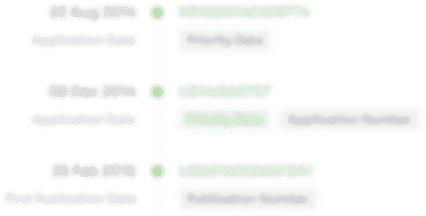
- R&D Engineer
- R&D Manager
- IP Professional
- Industry Leading Data Capabilities
- Powerful AI technology
- Patent DNA Extraction
Browse by: Latest US Patents, China's latest patents, Technical Efficacy Thesaurus, Application Domain, Technology Topic, Popular Technical Reports.
© 2024 PatSnap. All rights reserved.Legal|Privacy policy|Modern Slavery Act Transparency Statement|Sitemap|About US| Contact US: help@patsnap.com