Convolutional neural network hardware acceleration method of parallel computing unit
A convolutional neural network and parallel computing technology, applied to biological neural network models, computer components, calculations, etc., can solve problems such as reducing the amount of calculation, inappropriate calculation parameters, and not considering the generality of the model parameter network structure, etc., to achieve The effect of significant effect and high inference speed
- Summary
- Abstract
- Description
- Claims
- Application Information
AI Technical Summary
Problems solved by technology
Method used
Image
Examples
Embodiment Construction
[0029] The present invention will be further described below with reference to the accompanying drawings and embodiments.
[0030] Please refer to figure 1 , the present invention provides a convolutional neural network hardware acceleration method based on static quantization, hierarchical quantization and parallel computing units, comprising the following steps:
[0031] Step S1: the trained convolutional neural network model is quantified according to the method of static quantization, hierarchical quantization;
[0032] In this embodiment, specifically, step S1 is specifically:
[0033] Step S11: First, extract parameters from the model, visualize them as a histogram, and observe the distribution of parameters. figure 2 and image 3 The distributions of the example first convolutional layer and second convolutional layer are drawn respectively in . From the figure, we can see that most of the weight parameters of each layer are concentrated in a certain interval. For ...
PUM
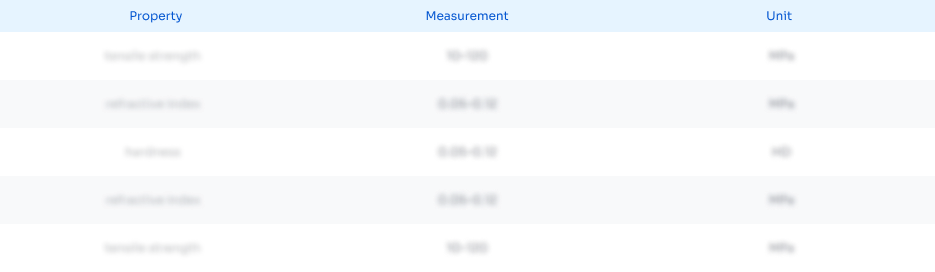
Abstract
Description
Claims
Application Information
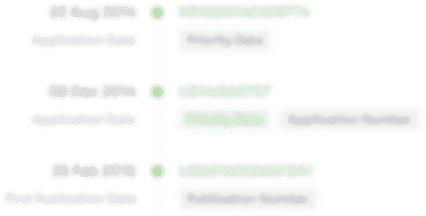
- R&D Engineer
- R&D Manager
- IP Professional
- Industry Leading Data Capabilities
- Powerful AI technology
- Patent DNA Extraction
Browse by: Latest US Patents, China's latest patents, Technical Efficacy Thesaurus, Application Domain, Technology Topic.
© 2024 PatSnap. All rights reserved.Legal|Privacy policy|Modern Slavery Act Transparency Statement|Sitemap