All-weather solar radiation remote sensing forecasting method under deep learning support
A technology of solar radiation and deep learning, which is applied in the field of remote sensing forecasting, can solve the problems that the absolute numerical accuracy of solar radiation and the distribution of spatial and temporal characteristics cannot be taken into account at the same time, and achieve the effect of superior anti-interference ability, strong robustness, and high precision
- Summary
- Abstract
- Description
- Claims
- Application Information
AI Technical Summary
Problems solved by technology
Method used
Image
Examples
Embodiment 1
[0066] like figure 1 and figure 2 The present embodiment as shown discloses an all-weather solar radiation remote sensing forecasting method supported by deep learning, which includes the following steps:
[0067] S1. Obtain time series multispectral remote sensing data; it should be noted that the time series multispectral remote sensing data here includes continuous time series multispectral remote sensing data and instantaneous time series multispectral remote sensing data.
[0068] S2. Perform data preprocessing on the acquired time series multispectral remote sensing data to obtain a preprocessed time series multispectral remote sensing data set;
[0069] S3. Input the acquired preprocessed time series multispectral remote sensing data set into the ConvLSTM network model to obtain instantaneous solar shortwave radiation prediction data or multi-time scale solar shortwave radiation prediction; the ConvLSTM network model obtains normalized solar shortwave radiation data, ...
Embodiment 2
[0113] In this embodiment, there are two parallel routes, which are:
[0114] 1. Instantaneous solar shortwave radiation prediction supported by deep learning technology;
[0115] 2. Multi-time scale solar shortwave radiation prediction supported by deep learning technology;
[0116] like figure 1 As shown: the first technical route in this embodiment, that is, the instantaneous solar shortwave radiation prediction supported by deep learning technology, the technical process is as follows:
[0117] Taking the Sunflower 8 multi-spectral optical remote sensing satellite as an example, the multi-spectral optical satellite remote sensing data of continuous time series are firstly obtained. Three types of raw data of zenith angle SOZ (Solar of Zenith) data and solar shortwave radiation data;
[0118] Data preprocessing is carried out on the acquired remote sensing data. The data preprocessing includes two parts: 1) Data quality control, checking the quality of the obtained data,...
PUM
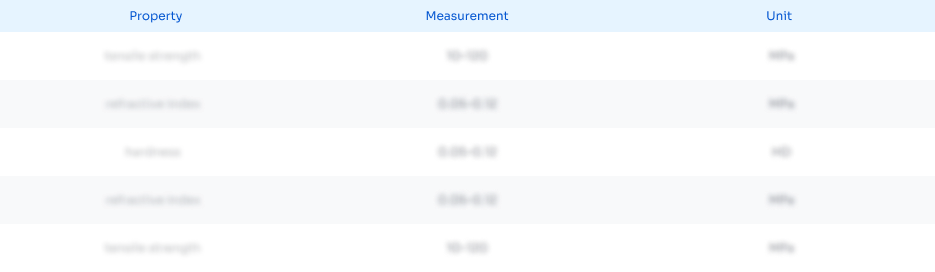
Abstract
Description
Claims
Application Information
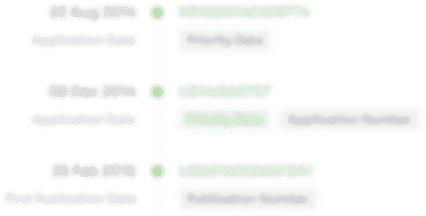
- Generate Ideas
- Intellectual Property
- Life Sciences
- Materials
- Tech Scout
- Unparalleled Data Quality
- Higher Quality Content
- 60% Fewer Hallucinations
Browse by: Latest US Patents, China's latest patents, Technical Efficacy Thesaurus, Application Domain, Technology Topic, Popular Technical Reports.
© 2025 PatSnap. All rights reserved.Legal|Privacy policy|Modern Slavery Act Transparency Statement|Sitemap|About US| Contact US: help@patsnap.com