Defect detection method based on cyclic generative adversarial network and structural similarity
A technology for structural similarity and defect detection, applied in biological neural network models, neural learning methods, neural architectures, etc., can solve the problems of large subjectivity, high detection error rate, poor detection effect, etc., and achieve fast model convergence speed , high detection accuracy, and the effect of improving efficiency
- Summary
- Abstract
- Description
- Claims
- Application Information
AI Technical Summary
Problems solved by technology
Method used
Image
Examples
Embodiment 1
[0082] In this embodiment, the computer environment is as follows, CPU: Intel(R) Xeon(R) CPU E5-2620 v4@2.10GHz, GPU: GeForce GTX 1080Ti, Memory: 128G, Python: 3.6.13, Pytorch: 1.7.1 .
[0083] 1. Data set production
[0084] The dataset is derived from Class6 ( Figure 5 middle a, Figure 5 in b) and Class7 ( Figure 5 middle c. Figure 5 In d), DAGM2007 is a common data set for industrial surface defect detection. There are 10 different categories. Each category is divided into training set and test set. All images are grayscale images in png format, 512 × 512 pixels.
[0085]In this embodiment, all training pictures and test pictures are resized to a size of 256×256 before being input into the model, and data standardization is performed with 0.5 as the mean and standard deviation, so as to speed up the convergence speed of the model. In addition, the training images are randomly cropped and randomly flipped to improve the robustness of the model.
[0086] The size of...
PUM
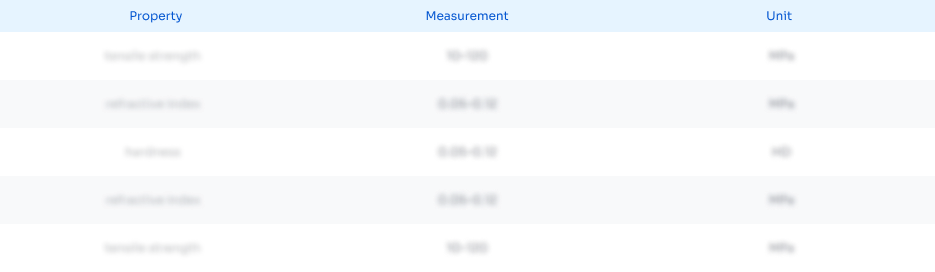
Abstract
Description
Claims
Application Information
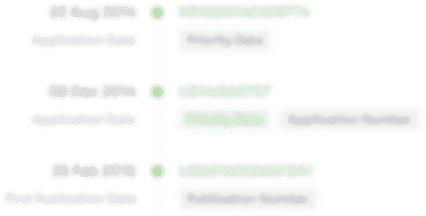
- R&D Engineer
- R&D Manager
- IP Professional
- Industry Leading Data Capabilities
- Powerful AI technology
- Patent DNA Extraction
Browse by: Latest US Patents, China's latest patents, Technical Efficacy Thesaurus, Application Domain, Technology Topic, Popular Technical Reports.
© 2024 PatSnap. All rights reserved.Legal|Privacy policy|Modern Slavery Act Transparency Statement|Sitemap|About US| Contact US: help@patsnap.com