Bus track similarity calculation method based on twin neural network
A technology of neural network and calculation method, applied in the direction of biological neural network model, neural learning method, calculation, etc., to achieve the effect of optimizing generalization ability, increasing feature distance, and reducing feature distance
- Summary
- Abstract
- Description
- Claims
- Application Information
AI Technical Summary
Problems solved by technology
Method used
Image
Examples
Embodiment 1
[0032] Embodiment 1: This embodiment provides a method for calculating the similarity of public transport tracks based on a twin neural network, and the method includes the following steps:
[0033] Step 1: Build a bus line set, select two bus lines from the bus line set, and perform line preprocessing on the two bus lines respectively; the line preprocessing includes the following steps:
[0034] S1. Obtain the spatial coordinate point sequence of the line
[0035] S2. Encrypt the nodes of the line;
[0036] S3. Convert the space coordinate point from the plane coordinate to the polar coordinate
[0037] S4. Complete the embedded vectorized expression of the data in combination with the neural network;
[0038] Step 2. Sample data organization
[0039] Translate the bus lines, take the translated lines as similar trajectories, and use them as positive samples, randomly sample from the line set to obtain negative samples, and obtain organized training samples, each of which...
Embodiment 2
[0050] Embodiment 2: This embodiment provides a method for calculating the similarity of public transport tracks based on a twin neural network. The following is a calculation process and method for similarity of public transport tracks through line preprocessing, sample data organization, model construction, model training and prediction. describe.
[0051] 1. Line preprocessing
[0052] Points, lines, and areas are the three core spatial data types, and bus lines are typical line spatial data types. In the field of GIS, a line is composed of an ordered sequence of points. In this embodiment, the Open Geospatial Consortium (OGC) (Open GIS Consortium) formulates a line-spatial data type vector structure represented by the Well-know Text text markup language, such as LineString(((0 ,0),(1,1))), where ((0,0)(1,1)) is the sequence of spatial coordinate points representing the line.
[0053] This embodiment encrypts the nodes of the line space data, and also considers the partic...
PUM
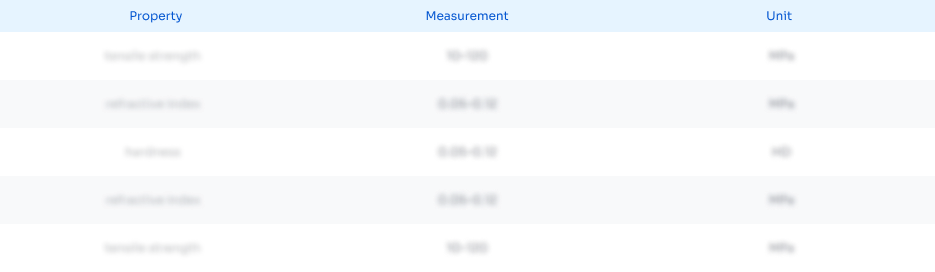
Abstract
Description
Claims
Application Information
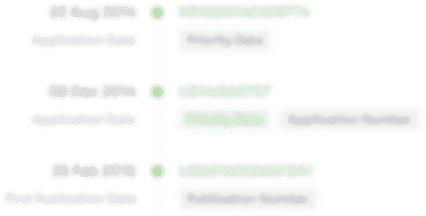
- R&D
- Intellectual Property
- Life Sciences
- Materials
- Tech Scout
- Unparalleled Data Quality
- Higher Quality Content
- 60% Fewer Hallucinations
Browse by: Latest US Patents, China's latest patents, Technical Efficacy Thesaurus, Application Domain, Technology Topic, Popular Technical Reports.
© 2025 PatSnap. All rights reserved.Legal|Privacy policy|Modern Slavery Act Transparency Statement|Sitemap|About US| Contact US: help@patsnap.com