Method for forecasting electric power system short-term load based on method for improving uttermost learning machine
A technology of short-term load forecasting and extreme learning, applied in neural learning methods, biological neural network models, etc., can solve the problems affecting the learning ability and generalization ability of the network prediction model, increasing the complexity of optimization calculations, and long training time, etc. question
- Summary
- Abstract
- Description
- Claims
- Application Information
AI Technical Summary
Problems solved by technology
Method used
Image
Examples
Embodiment Construction
[0024] The present invention will be described in further detail below in conjunction with the accompanying drawings and calculation examples.
[0025] see Figure 5 , read historical sample data: read the load data, week type and temperature data of the 2 years before the forecast date provided by the power department as historical sample data;
[0026] Historical data generally includes historical load data and temperature data, and the difference between these two types of data is one or several orders of magnitude. In order to make data of different magnitudes comparable and avoid calculation overflow, the data is first normalized before calculation.
[0027] In order to make the training process of the improved extreme learning machine network easy to converge, its input and output are usually normalized so that their values are in the interval [0, 1], set x t 、y t are the input and output normalized load values of the prediction network respectively, then
[0028...
PUM
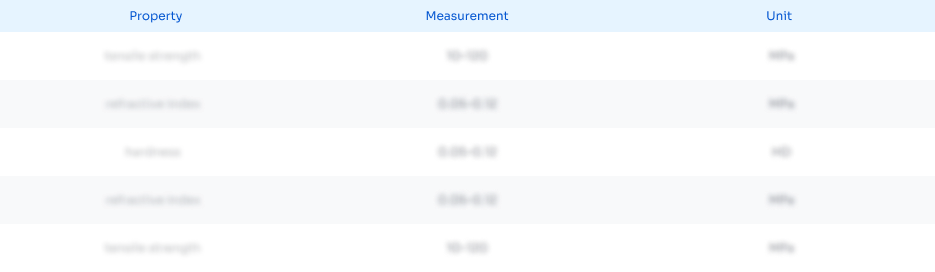
Abstract
Description
Claims
Application Information
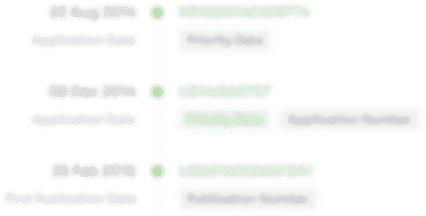
- R&D Engineer
- R&D Manager
- IP Professional
- Industry Leading Data Capabilities
- Powerful AI technology
- Patent DNA Extraction
Browse by: Latest US Patents, China's latest patents, Technical Efficacy Thesaurus, Application Domain, Technology Topic, Popular Technical Reports.
© 2024 PatSnap. All rights reserved.Legal|Privacy policy|Modern Slavery Act Transparency Statement|Sitemap|About US| Contact US: help@patsnap.com