Image classification method based on feature correlation of frequency domain direction
A classification method and correlation technology, applied in the field of image processing, can solve the problems of high computational complexity, insufficient sub-band frequency and direction division, and limited classification performance, so as to achieve reduced computational complexity, improved classification performance, and detailed division. Effect
- Summary
- Abstract
- Description
- Claims
- Application Information
AI Technical Summary
Problems solved by technology
Method used
Image
Examples
Embodiment Construction
[0023] refer to figure 1 , the specific implementation process of the present invention is as follows:
[0024] Step 1, select sample images of various textures, and divide these sample images into two data sets of training sample images and test sample images.
[0025] The present invention uses two image data sets for performance testing: Brodatz texture image and SAR texture image.
[0026] 1a) The selection method of the Brodatz texture image sample dataset is described as follows:
[0027] Select 77 types of uniform texture images in the standard Brodatz texture library as test data. These 77 types of textures are: D1, D3, D4, D5, D6, D8, D9, D11, D14, D16, D17, D18, D19, D20, D21, D22, D23, D24, D25, D26, D27, D28, D29, D32, D33, D34, D35, D36, D37, D38, D46, D47, D48, D49, D50, D51, D52, D53, D54, D55, D56, D57, D64, D65, D66, D68, D74, D75, D76, D77, D78, D79, D80, D81, D82, D83, D84, D85, D87, D88, D92, D93, D94, D95, D96, D98, D100, D101, D102, D103, D104, D105, ...
PUM
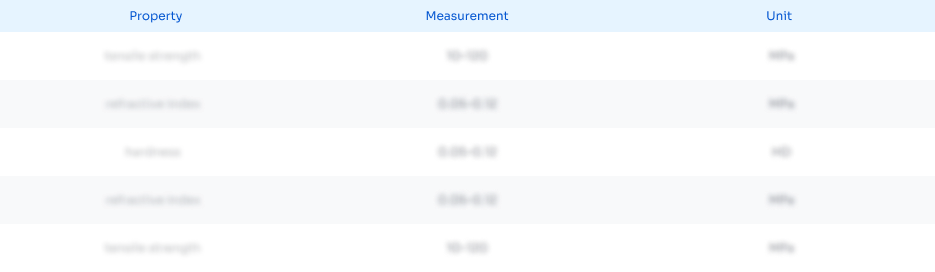
Abstract
Description
Claims
Application Information
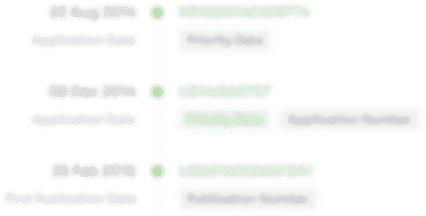
- R&D Engineer
- R&D Manager
- IP Professional
- Industry Leading Data Capabilities
- Powerful AI technology
- Patent DNA Extraction
Browse by: Latest US Patents, China's latest patents, Technical Efficacy Thesaurus, Application Domain, Technology Topic.
© 2024 PatSnap. All rights reserved.Legal|Privacy policy|Modern Slavery Act Transparency Statement|Sitemap