Semi-supervised neighborhood discrimination analysis method for face recognition
A technology of discriminant analysis and face recognition, applied in the field of image processing, which can solve the problems of insufficient labeled data, money-consuming and time-consuming, etc.
- Summary
- Abstract
- Description
- Claims
- Application Information
AI Technical Summary
Problems solved by technology
Method used
Image
Examples
Embodiment Construction
[0021] The invention provides a semi-supervised neighborhood discriminant analysis (SSNDA) method for face recognition. Combine below Figure 1-7 and different algorithms, the present invention will be further described based on spectrogram theory.
[0022] Spectral graph theory for dimensionality reduction
[0023] In classification problems, the training sample set can be expressed as a matrix X=[x 1 , x 2 ,...,x N ], x i ∈R M , where N is the number of samples, M is the feature dimension, and x i is the jth face sample, x i ∈R M Indicates the face sample x i belongs to the M-dimensional real number space. In a supervised learning problem, a sample x i The class label of c i ∈{1,2,...,n c}, where n c is the number of classes, N j is the number of samples belonging to the jth class.
[0024] Construct an undirected weighted graph G={X, A} using the graph-based dimensionality reduction method [2,3], where the vertex set is X, and the adjacency matrix or weight ...
PUM
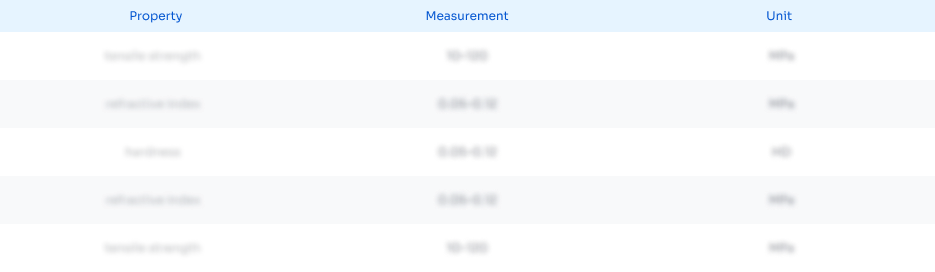
Abstract
Description
Claims
Application Information
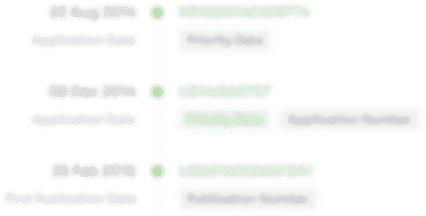
- R&D
- Intellectual Property
- Life Sciences
- Materials
- Tech Scout
- Unparalleled Data Quality
- Higher Quality Content
- 60% Fewer Hallucinations
Browse by: Latest US Patents, China's latest patents, Technical Efficacy Thesaurus, Application Domain, Technology Topic, Popular Technical Reports.
© 2025 PatSnap. All rights reserved.Legal|Privacy policy|Modern Slavery Act Transparency Statement|Sitemap|About US| Contact US: help@patsnap.com