Combination learning super-resolution method based on dual constraint
A dual-constrained, super-resolution technology, applied in the field of image processing, can solve the problem that the feature space of high-resolution image blocks is not optimal, and the impact is not considered.
- Summary
- Abstract
- Description
- Claims
- Application Information
AI Technical Summary
Problems solved by technology
Method used
Image
Examples
Embodiment Construction
[0035] refer to figure 1 , the joint learning super-resolution method based on dual constraints mainly includes two stages: the training set generation stage and the image super-resolution stage.
[0036] 1. Training set generation stage
[0037] Step 1. Simulate the image degradation process, build a feature library, and generate a training set.
[0038] 1a) Collect a large number of high-resolution gray-scale natural images, and generate corresponding low-resolution images for each high-resolution image through 4×4 average blurring and 3 times downsampling;
[0039] 1b) For each low-resolution image, use bicubic interpolation to enlarge by 2 times to obtain an interpolated image, and use the following 4 convolution kernels:
[0040] f 1 = [-1, 0, 1], f 2 = [-1, 0, 1] T , f 3 = [1, 0, -2, 0, 1], f 4 =[1, 0, -2, 0, 1] T Carry out convolution with the interpolation image and the corresponding original high-resolution image respectively, and generate the first-order grad...
PUM
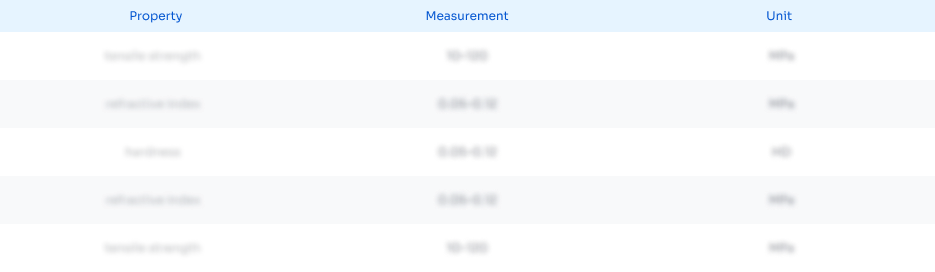
Abstract
Description
Claims
Application Information
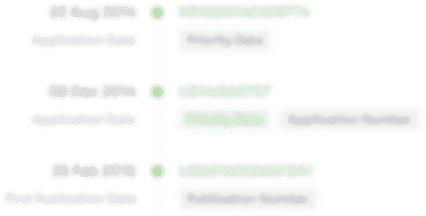
- R&D Engineer
- R&D Manager
- IP Professional
- Industry Leading Data Capabilities
- Powerful AI technology
- Patent DNA Extraction
Browse by: Latest US Patents, China's latest patents, Technical Efficacy Thesaurus, Application Domain, Technology Topic, Popular Technical Reports.
© 2024 PatSnap. All rights reserved.Legal|Privacy policy|Modern Slavery Act Transparency Statement|Sitemap|About US| Contact US: help@patsnap.com