Semi-supervised learning-based recommendation system shilling attack detection method
A semi-supervised learning and attack detection technology, which is applied in the field of information security and can solve the problems of large errors, inability to determine the identity, and no use of the attack detection method.
- Summary
- Abstract
- Description
- Claims
- Application Information
AI Technical Summary
Problems solved by technology
Method used
Image
Examples
Embodiment Construction
[0058] A semi-supervised learning based recommendation system attack detection method of the present invention comprises the following stages:
[0059] 1) The preprocessing stage of troll attack detection; in this stage, the troll attack detection indicators of the marked and unmarked datasets are obtained by preprocessing the data of the marked data set and the unmarked data set, and then the initial naive attack detection index is trained on the marked data set Bayesian classifier;
[0060] If the category of the user is known, it belongs to the labeled data set L, otherwise it belongs to the unlabeled data set U; L={(u1,c1),(u2,c2),...,(u|L|,c|L| )} is a labeled data set, (u1, u2,,..., u|L|) represents a user set, (c1, c2,,..., c|L|) represents a type set of the user, and U={u' 1, u'2,..., u'|U|} are unlabeled data sets;
[0061] 2) EM-λ algorithm stage; in this stage, a stable classifier is continuously iteratively obtained through the EM-λ algorithm, and finally the typ...
PUM
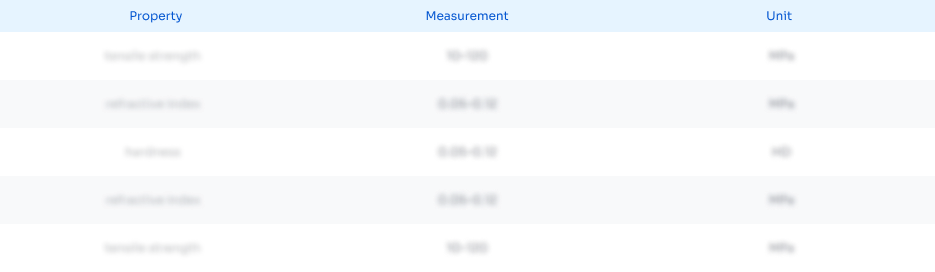
Abstract
Description
Claims
Application Information
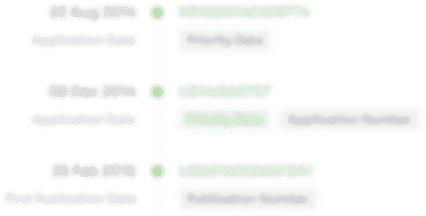
- Generate Ideas
- Intellectual Property
- Life Sciences
- Materials
- Tech Scout
- Unparalleled Data Quality
- Higher Quality Content
- 60% Fewer Hallucinations
Browse by: Latest US Patents, China's latest patents, Technical Efficacy Thesaurus, Application Domain, Technology Topic, Popular Technical Reports.
© 2025 PatSnap. All rights reserved.Legal|Privacy policy|Modern Slavery Act Transparency Statement|Sitemap|About US| Contact US: help@patsnap.com