Satellite clock error prediction method based on empirical mode decomposition (EMD) model and generalized autoregressive conditional heteroskedasticity (GARCH) model
A satellite clock error and clock error technology, applied in character and pattern recognition, instruments, computer parts, etc., can solve problems such as difficulty in improving forecast accuracy, and achieve improved clock error forecast accuracy, prediction accuracy, and pattern recognition difficulties. Effect
- Summary
- Abstract
- Description
- Claims
- Application Information
AI Technical Summary
Problems solved by technology
Method used
Image
Examples
specific Embodiment approach 1
[0017] Specific implementation mode 1: Combination figure 1 To describe this embodiment, this embodiment includes the following specific steps:
[0018] Step 1: Obtain historical clock error data, and perform data correction preprocessing to obtain satellite clock error data;
[0019] Step 2: Decompose the empirical mode of the satellite clock error data; use EMD to decompose the satellite clock error data into a series of eigenmode function and residual function components with different frequency components; the frequency of the eigenmode function has From high to low, after removing the high frequency part, the eigenmode function is summed to obtain the random term part of the satellite clock error data, and the trend term of the satellite clock error data is obtained through the residual function, and the residual function reflects the satellite The trend item part of the clock error data;
[0020] Step 3: Predict the trend item of the satellite clock error data. The trend of t...
specific Embodiment approach 2
[0022] Embodiment 2: If there are abnormal points, no data segments, or data abnormalities in the clock error history data acquired in step one of specific embodiment 1, the abnormalities are corrected, and for abnormal points, the abnormalities are deleted. Point, and then merge into no data section; for no data section, use polynomial interpolation method to get the data of no data section; for data jump, use sliding window to detect jump, for jump data, take the front-end data and use it Segment data is used to predict the clock error. The other composition and connection relationship are the same as in the first embodiment.
[0023] Step 2: Before the EMD analysis of the satellite clock error data, determine whether the following conditions are met at the same time: a. The analyzed data contains at least two extreme points, a maximum value and a minimum value; b. According to the two adjacent extreme points The time distance can define the characteristic time scale; c. If th...
specific Embodiment approach 3
[0025] Specific embodiment three: the sub-steps included in step two in specific embodiment one are as follows:
[0026] Substep 1. Obtain the maximum value y(t) from the satellite clock error data y(t) u ) And the minimum value y(t v ), where u = 1, 2,..., N u , V=1, 2,..., N v , N u Is the number of maxima, N v Is the number of minimum values; the upper and lower envelopes y of the maximum point and the minimum point are constructed using cubic spline function respectively u (t) and y v (t), calculate the mean of the two envelopes
[0027] m 1 = 1 2 ( y u ( t ) + y v ( t ) ) ; - - - ( 1 )
[0028] Sub-step 2, judge h 1 =y(t)-m 1 Whether the following two conditions are met at the same time as the IMF:
[0029] a. The number of data extreme points is equal to or one difference from the number of zero points;
[0030] b. The local mean of the upper envelope defined by the maximum value and the lower envelope defined b...
PUM
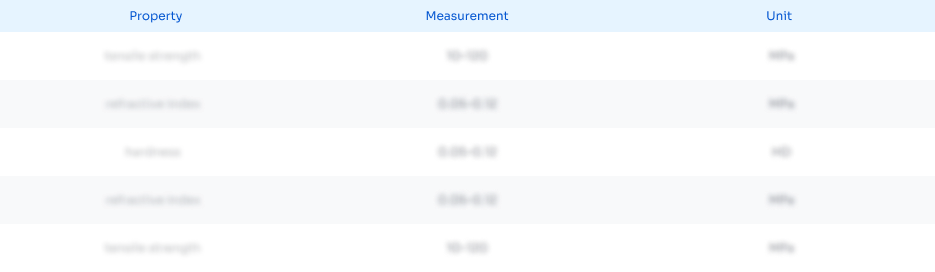
Abstract
Description
Claims
Application Information
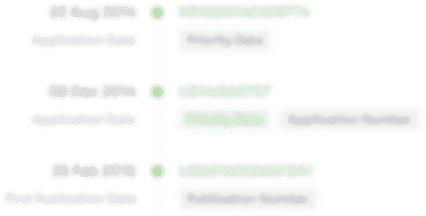
- R&D Engineer
- R&D Manager
- IP Professional
- Industry Leading Data Capabilities
- Powerful AI technology
- Patent DNA Extraction
Browse by: Latest US Patents, China's latest patents, Technical Efficacy Thesaurus, Application Domain, Technology Topic.
© 2024 PatSnap. All rights reserved.Legal|Privacy policy|Modern Slavery Act Transparency Statement|Sitemap