Working fluid level prediction-based optimization method for stroke frequency of submersible reciprocating oil pumping unit
An optimization method and dynamic fluid surface technology, applied in the direction of instruments, self-adaptive control, control/regulation systems, etc., can solve the problems of submersible reciprocating pumping units without stroke optimization control methods, and can not adjust linear motors, etc., to achieve Significant energy-saving effect, improved control automation, and simple algorithm
- Summary
- Abstract
- Description
- Claims
- Application Information
AI Technical Summary
Problems solved by technology
Method used
Image
Examples
specific Embodiment approach 1
[0017] Specific implementation mode one: combine figure 1 Describe this implementation mode, this implementation mode is realized through the following steps:
[0018] Step 1, normalization processing of the time series of the dynamic liquid surface;
[0019] Step 2. Reconstruct the sample space of the dynamic liquid surface time series after step 1 normalization;
[0020] Step 3, establishing a support vector dynamic liquid level prediction model, and performing regression prediction of the dynamic liquid level time series;
[0021] Step 4. Determine the economical objective function of stroke optimization
[0022] Taking the parameters or functions related to the economy of the operation of the submersible reciprocating pumping unit as parameters, the function model of stroke times and economic indicators is established, and according to the strength of the influence of each parameter in the economic function on the function, Simplify the economic function to obtain the...
specific Embodiment approach 2
[0026] Specific implementation mode two: the normalization processing process of the dynamic liquid surface time series in the step one of the present implementation mode:
[0027] use the formula Change the sample data of dynamic liquid level and stroke times to the same order of magnitude range, normalize the original data of dynamic liquid level and linear motor stroke times to between [-1, 1],
[0028] In the formula: For normalized data, x i is the original sample data, x max is the maximum value in the time series, x min is the minimum value in the time series.
[0029] When the support vector machine model predicts, it focuses on modifying the weights corresponding to variables with large values, which will affect the weight training corresponding to variables with small values. Therefore, variables with large values have a greater impact on the output deviation, while dynamic The change range of the liquid level data is large, and the order of magnitude differ...
specific Embodiment approach 3
[0030] Specific implementation mode three: the reconstruction process of the dynamic liquid surface time series sample space in step two of this embodiment: based on the normalized time series of the dynamic liquid surface and stroke times, construct a support vector machine training sample, and input For the reconstruction of the sample space, the input and output matrices are
[0031] Formula one:
[0032] Formula two: y 1 y 2 . . . y n - m = ...
PUM
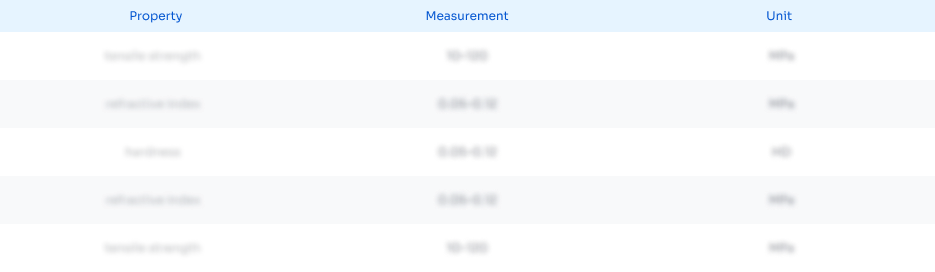
Abstract
Description
Claims
Application Information
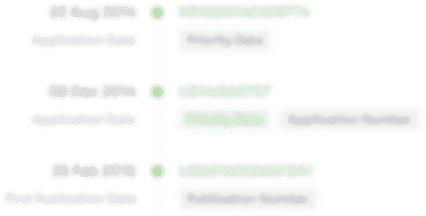
- Generate Ideas
- Intellectual Property
- Life Sciences
- Materials
- Tech Scout
- Unparalleled Data Quality
- Higher Quality Content
- 60% Fewer Hallucinations
Browse by: Latest US Patents, China's latest patents, Technical Efficacy Thesaurus, Application Domain, Technology Topic, Popular Technical Reports.
© 2025 PatSnap. All rights reserved.Legal|Privacy policy|Modern Slavery Act Transparency Statement|Sitemap|About US| Contact US: help@patsnap.com