Object type identification method combining plurality of interest point testers
An interest point detection and interest point technology, applied in the fields of image understanding, computer vision, and pattern recognition, can solve problems such as poor robustness, complex model, and excessive supervision, achieving good average effect, simple practice, and reduced supervision. Effect
- Summary
- Abstract
- Description
- Claims
- Application Information
AI Technical Summary
Problems solved by technology
Method used
Image
Examples
specific Embodiment
[0038] Resize the images so that each image contains approximately 40,000 pixels (aspect ratio preserved). Because the SIFT descriptor is the most popular and effective descriptor, and most existing related methods use 128-dimensional SIFT vectors to describe interest points. So preferred embodiments also use it to delineate points of interest. Each time 60% of the images are selected to form a new training subset. 60 interest points are randomly selected from each image, and k-means is used to construct a visual dictionary of members. Because of the inherent randomness of the k-means algorithm, it is equivalent to using different clusterers when forming different member dictionaries. In most studies related to the “bag-of-words” model, the size of the visual dictionary is between 100 and 1000, so this parameter is set to a middle value of 500. Linear SVM (Support Vector Machine) learns a classifier based on the quantized vector set of each member dictionary. This process ...
PUM
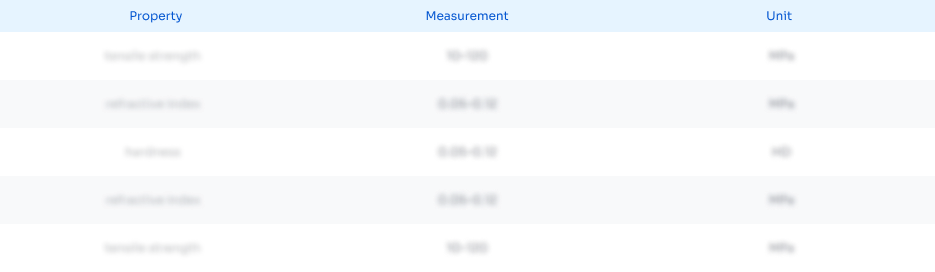
Abstract
Description
Claims
Application Information
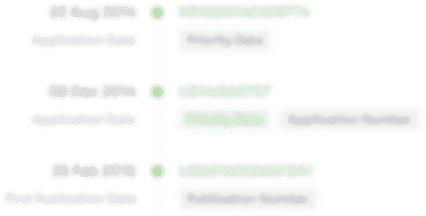
- R&D Engineer
- R&D Manager
- IP Professional
- Industry Leading Data Capabilities
- Powerful AI technology
- Patent DNA Extraction
Browse by: Latest US Patents, China's latest patents, Technical Efficacy Thesaurus, Application Domain, Technology Topic, Popular Technical Reports.
© 2024 PatSnap. All rights reserved.Legal|Privacy policy|Modern Slavery Act Transparency Statement|Sitemap|About US| Contact US: help@patsnap.com