SAM weighted KEST hyperspectral anomaly detection algorithm
An anomaly detection and hyperspectral technology, applied in the field of hyperspectral image processing, can solve the problems of not using correlation well, and achieve the effect of suppressing abnormal data
- Summary
- Abstract
- Description
- Claims
- Application Information
AI Technical Summary
Problems solved by technology
Method used
Image
Examples
Embodiment Construction
[0039] The SAM weighted KEST hyperspectral anomaly detection algorithm of the present invention, firstly, derives the SAM weighted KEST algorithm; secondly, uses a double rectangular window to calculate its SKEST value for each pixel in the hyperspectral image, and performs threshold segmentation to detect abnormal points.
[0040] 1. SAM weighted KEST algorithm:
[0041] It can be seen from the formula [2] that the target and background correlation matrix , The weight of each pixel in is equal, but if the background data is ill-conditioned distribution, the number of abnormal points in the background data or the number of background points in the target data is large, , The distribution of target and background data cannot be fully described, and the target detection efficiency is low. Therefore this patent proposes a kind of SAM weighted KEST algorithm, this method uses , The spectral angle matching (SAM) of each pixel spectral vector and the data center vector ...
PUM
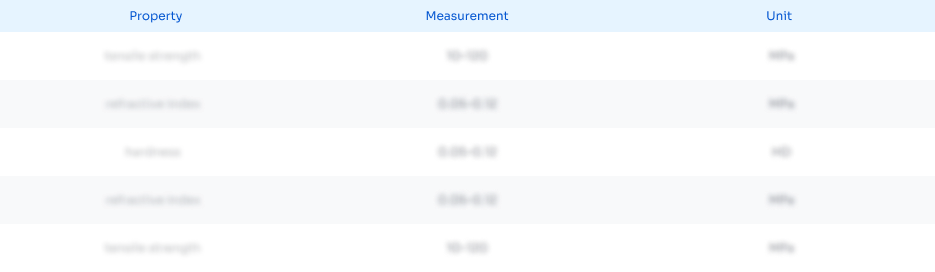
Abstract
Description
Claims
Application Information
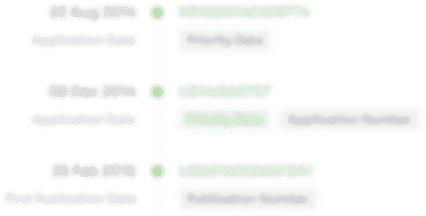
- R&D Engineer
- R&D Manager
- IP Professional
- Industry Leading Data Capabilities
- Powerful AI technology
- Patent DNA Extraction
Browse by: Latest US Patents, China's latest patents, Technical Efficacy Thesaurus, Application Domain, Technology Topic, Popular Technical Reports.
© 2024 PatSnap. All rights reserved.Legal|Privacy policy|Modern Slavery Act Transparency Statement|Sitemap|About US| Contact US: help@patsnap.com