A prediction method for crankshaft fatigue life based on genetic nerve network
A fatigue life prediction and fatigue life technology, applied in the direction of biological neural network model, gene model, etc., can solve the problems of crankshaft test damage and long test time.
- Summary
- Abstract
- Description
- Claims
- Application Information
AI Technical Summary
Problems solved by technology
Method used
Image
Examples
Embodiment Construction
[0065] Below in conjunction with embodiment the method of the present invention is described in detail. The present invention is not limited to the following examples, and any design idea utilizing the present invention falls within the protection scope of the present invention.
[0066] First, the original test data of 100 DC resonant crankshaft fatigue life testing machines, where p1-p10 is the crankshaft natural frequency unit (Hz) collected every 6 seconds within 1 minute, and the fatigue life is the test cycle number unit (times), See Table 1.
[0067] Table 1 The original data of the DC resonance crankshaft fatigue life testing machine in the first half of 2011
[0068]
[0069] Normalize the original data in Table 1 to build a training sample set (a 1 ...a 100 ), where each training sample a i (1≤i≤100) contains 10 inputs and 1 crankshaft fatigue life history test value, such as Figure 4 shown. For example, the first training sample a in the training sample se...
PUM
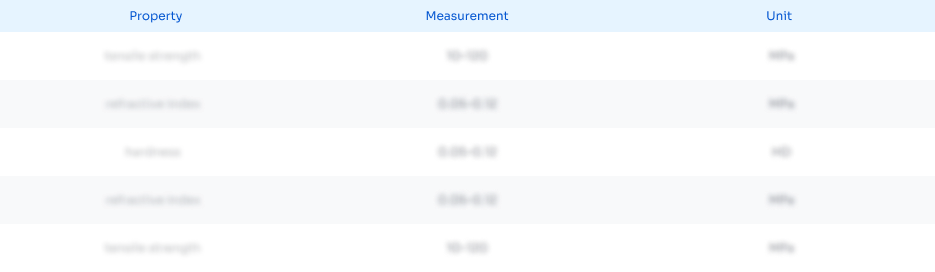
Abstract
Description
Claims
Application Information
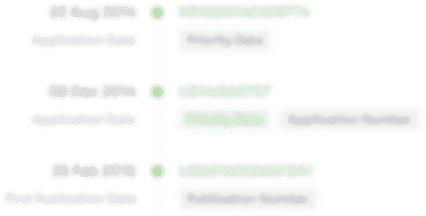
- R&D Engineer
- R&D Manager
- IP Professional
- Industry Leading Data Capabilities
- Powerful AI technology
- Patent DNA Extraction
Browse by: Latest US Patents, China's latest patents, Technical Efficacy Thesaurus, Application Domain, Technology Topic.
© 2024 PatSnap. All rights reserved.Legal|Privacy policy|Modern Slavery Act Transparency Statement|Sitemap