Mel frequency cepstrum coefficient (MFCC) underwater target feature extraction and recognition method
An underwater target and feature extraction technology, applied in character and pattern recognition, instruments, computer parts, etc., can solve problems such as difficult qualitative description of target characteristics
- Summary
- Abstract
- Description
- Claims
- Application Information
AI Technical Summary
Problems solved by technology
Method used
Image
Examples
Embodiment 1
[0108] Simulation model: the line spectrum is generated by a periodic signal, and the environmental noise is generated by Gaussian white noise with zero mean value. f i Take 30Hz, 47Hz, 65Hz, 89Hz, 110Hz, 247Hz, 580Hz respectively, signal-to-noise ratio where σ 2 is the variance of Gaussian white noise, sampling frequency f s 10KHz, the number of samples for each class is 4000, the number of samples for each class is 100, and the signal-to-noise ratio is -14dB.
[0109] Table 1 Recognition rate of two types of target signals with different SNR
[0110]
Embodiment 2
[0112] The three types of underwater targets A, B, and C have a sampling frequency of 22.5KHz, and the number of samples of each type is 48. In the first step of frame processing, the length of each frame is 512, and the number of repeated data between frames is 256. The number of triangular filter banks is 36, and the feature dimension for DCT transformation is 24, so the final combined feature dimension is 66. The clustering classifier based on the mixed Gaussian model of the EM algorithm, the initial value setting of the mean and variance is set by the K-means method, and the condition for the classifier to stop iterating is that the sum of the squares of the parameter errors of the two previous and subsequent times does not exceed 0.01. Figures 2, 3, and 4 are the underwater targets A, B, and C, respectively, after passing through the triangular filter bank and passing through the linear differential operator (Sobel operator and Laplacian operator in the t direction and f ...
PUM
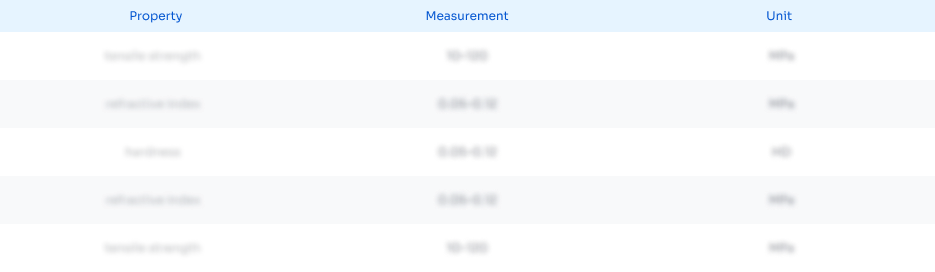
Abstract
Description
Claims
Application Information
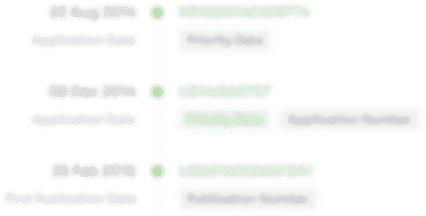
- R&D
- Intellectual Property
- Life Sciences
- Materials
- Tech Scout
- Unparalleled Data Quality
- Higher Quality Content
- 60% Fewer Hallucinations
Browse by: Latest US Patents, China's latest patents, Technical Efficacy Thesaurus, Application Domain, Technology Topic, Popular Technical Reports.
© 2025 PatSnap. All rights reserved.Legal|Privacy policy|Modern Slavery Act Transparency Statement|Sitemap|About US| Contact US: help@patsnap.com