2D seismic data all-horizon automatic tracking method based on unsupervised classification
A seismic data and automatic tracking technology, applied in the field of seismic exploration data interpretation, can solve problems such as large impact, training samples cannot cover all conditions, approximation degree problem solving method solution accuracy, etc.
- Summary
- Abstract
- Description
- Claims
- Application Information
AI Technical Summary
Problems solved by technology
Method used
Image
Examples
Embodiment Construction
[0037] The following description with reference to the accompanying drawings is provided to assist in a comprehensive understanding of embodiments of the present invention as defined by the claims and their equivalents. Various specific details are included to aid in understanding but are to be regarded as exemplary only. Accordingly, those of ordinary skill in the art will recognize that various changes and modifications of the embodiments described herein can be made without departing from the scope and spirit of the invention. Also, descriptions of well-known functions and constructions are omitted for clarity and conciseness.
[0038] figure 1 It is a flow chart of the method for automatic tracking of full horizons of two-dimensional seismic data based on unsupervised classification according to the present invention.
[0039] refer to figure 1 , in step 101, read in 2D seismic data and control horizon data. Wherein, the two-dimensional seismic data may include the sta...
PUM
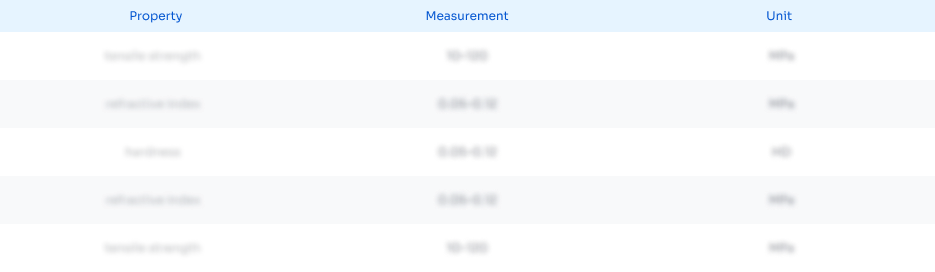
Abstract
Description
Claims
Application Information
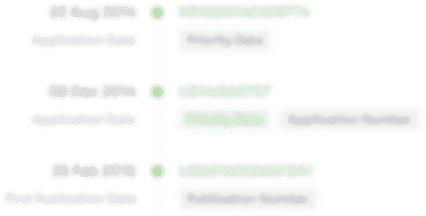
- R&D Engineer
- R&D Manager
- IP Professional
- Industry Leading Data Capabilities
- Powerful AI technology
- Patent DNA Extraction
Browse by: Latest US Patents, China's latest patents, Technical Efficacy Thesaurus, Application Domain, Technology Topic, Popular Technical Reports.
© 2024 PatSnap. All rights reserved.Legal|Privacy policy|Modern Slavery Act Transparency Statement|Sitemap|About US| Contact US: help@patsnap.com