Implementation method of metropolis-hastings mutation particle swarm resampling particle filter
A particle filter and implementation method technology, applied in impedance networks, adaptive networks, electrical components, etc., can solve the problems of increasing the risk of divergence, not considering the state probability density distribution, etc.
- Summary
- Abstract
- Description
- Claims
- Application Information
AI Technical Summary
Problems solved by technology
Method used
Image
Examples
Embodiment Construction
[0035] The specific implementation process of the present invention will be described in detail below, and an example will be given in conjunction with a nonlinear system model.
[0036] The execution steps of MH variation particle swarm optimization resampling particle filter of the present invention are as follows:
[0037] Step 1: Initialize the particle collection Among them, 1≤i≤N, represents the i-th particle in the particle set, and N is the total number of particles; and the initial position of the particle is randomly set and initial velocity Among them, 1≤k≤K, represents the kth sampling point, and K is the total number of sampling points of the signal;
[0038] Step 2: According to the state transition function F of the system k (·), to predict the state of the particle, namely
[0039] Step 3: Calculate the likelihood distribution value of the particles using the observation equation of the system: where Y k is the observed value, Y pred Observations p...
PUM
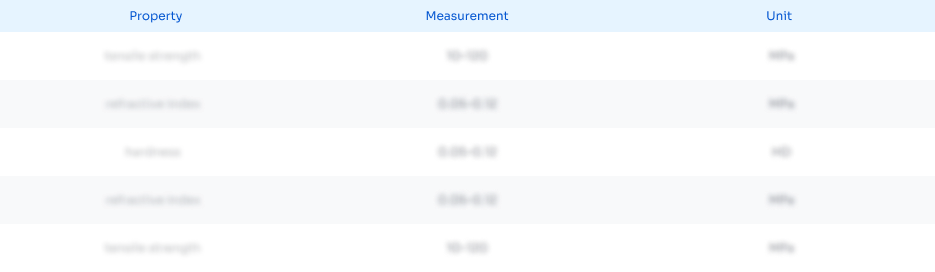
Abstract
Description
Claims
Application Information
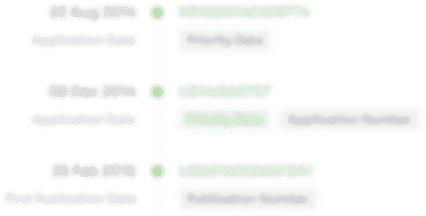
- R&D Engineer
- R&D Manager
- IP Professional
- Industry Leading Data Capabilities
- Powerful AI technology
- Patent DNA Extraction
Browse by: Latest US Patents, China's latest patents, Technical Efficacy Thesaurus, Application Domain, Technology Topic, Popular Technical Reports.
© 2024 PatSnap. All rights reserved.Legal|Privacy policy|Modern Slavery Act Transparency Statement|Sitemap|About US| Contact US: help@patsnap.com