Image content retrieval system and image content sparse learning method thereof
A technology of image content and retrieval system, applied in special data processing applications, instruments, electronic digital data processing, etc., can solve the problem that image semantic information cannot be fully and effectively expressed, and achieve the effect of easy programming
- Summary
- Abstract
- Description
- Claims
- Application Information
AI Technical Summary
Problems solved by technology
Method used
Image
Examples
Embodiment Construction
[0015] The present invention will be further described below in conjunction with the accompanying drawings.
[0016] like Figure 1-2 As shown, in multi-instance learning, each sample is composed of a set of unlabeled feature vectors, each feature vector is called an example, and a labeled sample composed of a set of examples is called a bag. The problem is described as follows: Assume that each data in the training set is a bag (Bag, that is, a sample), each bag is composed of multiple examples, each training bag has a concept label, but the example has no concept label. If at least one example in a bag is positive, the bag is marked as positive; if none of the examples in the bag is positive, the bag is marked as negative. The learning system learns the training set composed of multiple packages to predict the concept labels of packages outside the training set as correctly as possible. When using multi-instance learning in CBIR, the image must first be represented as a mu...
PUM
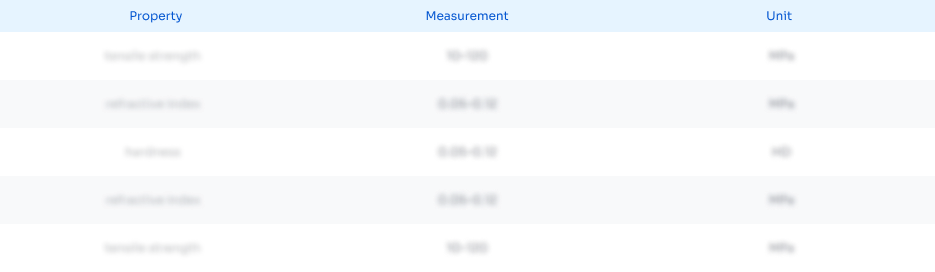
Abstract
Description
Claims
Application Information
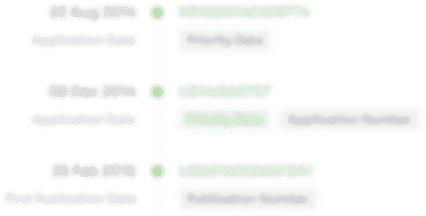
- R&D
- Intellectual Property
- Life Sciences
- Materials
- Tech Scout
- Unparalleled Data Quality
- Higher Quality Content
- 60% Fewer Hallucinations
Browse by: Latest US Patents, China's latest patents, Technical Efficacy Thesaurus, Application Domain, Technology Topic, Popular Technical Reports.
© 2025 PatSnap. All rights reserved.Legal|Privacy policy|Modern Slavery Act Transparency Statement|Sitemap|About US| Contact US: help@patsnap.com