Hyperspectral image classification method based on image regular low-rank expression dimensionality reduction
A high-spectral image and low-rank representation technology, which is applied in the field of remote sensing image processing, can solve the problems that the useful information of hyperspectral image data cannot be well preserved, the influence of hyperspectral image noise is not considered at the same time, and the memory requirements of computing devices are large. , to achieve the effect of maintaining the spatial distribution characteristics, reducing the amount of calculation, and reducing the complexity of calculation
- Summary
- Abstract
- Description
- Claims
- Application Information
AI Technical Summary
Problems solved by technology
Method used
Image
Examples
Embodiment 1
[0027] The invention proposes a hyperspectral image classification method based on graph-regular low-rank representation and dimensionality reduction. At present, hyperspectral images are extremely important in military and civilian applications. However, because the rich spectral information of hyperspectral images contains a lot of redundant information, the huge amount of data also affects the classification efficiency and classification accuracy of hyperspectral images, so the dimensionality reduction of hyperspectral images is very important in the application of hyperspectral image classification. has a very important role. Aiming at the shortcomings of the existing hyperspectral image dimensionality reduction methods that do not maintain the spatial distribution characteristics of hyperspectral images well and efficiently mine the local manifold structure information of hyperspectral images, combined with mean shift pre-segmentation and graph regular low-rank representa...
Embodiment 2
[0073] Hyperspectral image classification method based on graph regular low-rank representation dimensionality reduction, same as embodiment 1
[0074] 1. Simulation conditions:
[0075] The simulation experiment uses the Indian Pine image acquired by the airborne visible light / infrared imaging spectrometer AVIRIS of NASA Jet Propulsion Laboratory in June 1992 in northwestern Indiana, such as figure 2 As shown, the image size is 145×145, with a total of 220 bands, and there are 200 bands for noise removal and atmospheric and water absorption bands, with a total of 16 types of ground object information. The main types of information are listed in Table 1.
[0076] Table 1: Class attributes for Indian Pine images
[0077] Category number
category
Number of samples
1
Alfalfa
46
2
Corn-notill
1428
3
Corn-mintill
830
4
corn
237
5
Grass-pasture
483
6
Grass-trees
730
7...
Embodiment 3
[0085] The hyperspectral image classification method based on graph regular low-rank representation for dimensionality reduction is the same as in Example 1, and the simulation data and conditions are the same as in Example 2.
[0086] As shown in Table 2, Table 2 is the classification accuracy rate of the hyperspectral image Indian Pine when different numbers of training samples are selected for each category. The results in Table 2 are the average of the classification results of 30 randomly selected training samples, MRLRR, The dimensions of PCA and KPCA are reduced to 30 dimensions, and the dimensions of LDA are reduced to 15 dimensions. In this example, 8 and 12 samples of each category are randomly selected as training samples, and the rest are used as test samples. As can be seen from Table 2, when there are very few training samples, the classification accuracy rate of the present invention is higher than other methods, and as the training sample book increases, the cl...
PUM
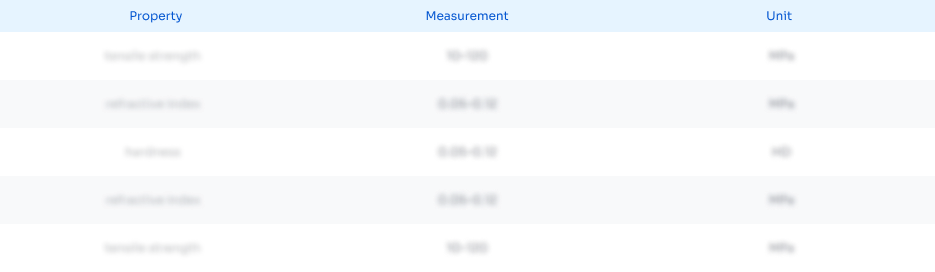
Abstract
Description
Claims
Application Information
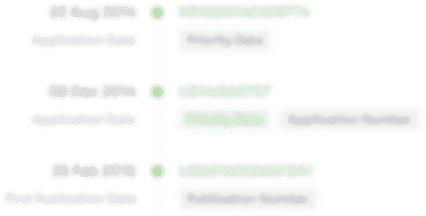
- R&D Engineer
- R&D Manager
- IP Professional
- Industry Leading Data Capabilities
- Powerful AI technology
- Patent DNA Extraction
Browse by: Latest US Patents, China's latest patents, Technical Efficacy Thesaurus, Application Domain, Technology Topic.
© 2024 PatSnap. All rights reserved.Legal|Privacy policy|Modern Slavery Act Transparency Statement|Sitemap