Video Anomaly Detection Method Based on Loop Graph Model
An anomaly detection and graph model technology, applied in the field of computer vision, which can solve the problems of over-fitting and under-fitting of video anomaly detection models
- Summary
- Abstract
- Description
- Claims
- Application Information
AI Technical Summary
Problems solved by technology
Method used
Image
Examples
Embodiment Construction
[0166] The implementation flow chart of the present invention is as figure 1 As shown, the specific implementation steps are as follows:
[0167] Step 1. Bottom-level feature extraction: For the recorded video, use the SIFT algorithm to extract two-dimensional image position information features for each frame image, obtain 128-dimensional direction parameters of several feature points, cluster these feature points, and construct BOW words bag form;
[0168] Step 2. Use the BOW bag of words of the video frame sequence as the document D, extract the topic semantic features through the LDA model, and obtain the topic feature matrix γ representing the topic features of each frame of image;
[0169] Step 3. Use the topic feature matrix γ as the observation of the loop HMM-LDA model to learn the parameters of the previous part of the loop HMM-LDA. By introducing an auxiliary variable u, the number of states in the latent state trajectory is a finite value, Use the method of dynam...
PUM
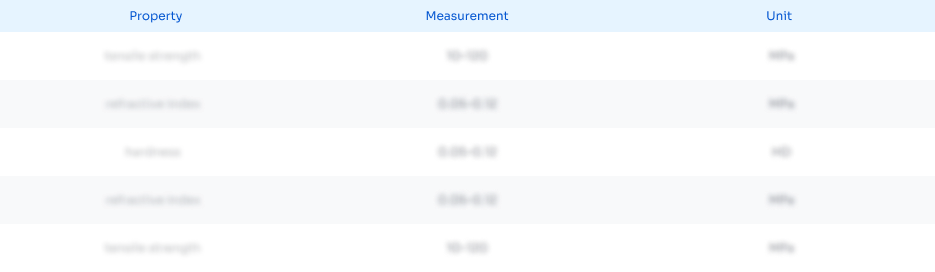
Abstract
Description
Claims
Application Information
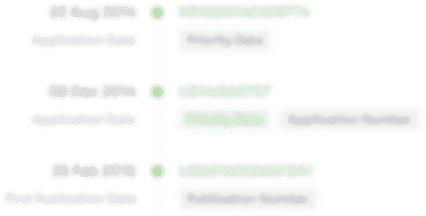
- R&D Engineer
- R&D Manager
- IP Professional
- Industry Leading Data Capabilities
- Powerful AI technology
- Patent DNA Extraction
Browse by: Latest US Patents, China's latest patents, Technical Efficacy Thesaurus, Application Domain, Technology Topic, Popular Technical Reports.
© 2024 PatSnap. All rights reserved.Legal|Privacy policy|Modern Slavery Act Transparency Statement|Sitemap|About US| Contact US: help@patsnap.com