Combination-kernel-function RVM (Relevance Vector Machine) hyperspectral classification method integrated with multi-scale morphological characteristics
A technology that combines kernel functions and morphological features, applied to instruments, character and pattern recognition, computer components, etc., can solve problems that have not been reported
- Summary
- Abstract
- Description
- Claims
- Application Information
AI Technical Summary
Problems solved by technology
Method used
Image
Examples
Embodiment Construction
[0079] The invention proposes a new combined kernel function RVM hyperspectral classification method that combines multi-scale morphological features. Using the method of the present invention to carry out real hyperspectral data classification experiments, the combined kernel function RVM classification method was compared with the traditional RVM classification method based solely on spectral features in terms of accuracy and sparseness.
[0080] The present invention will be further described in detail below in conjunction with the accompanying drawings and preferred embodiments. The computer hardware environment that described experiment adopts is Intel Core2 dual-core CPU, 1.58GHz / 3.25GB internal memory. The software environment is Microsoft Windows XP, Matlab R2008a. The algorithm described in the present invention is realized by MATLAB R2008a.
[0081]The correspondence between constituent elements in the claims and specific examples in the embodiments can be exemplif...
PUM
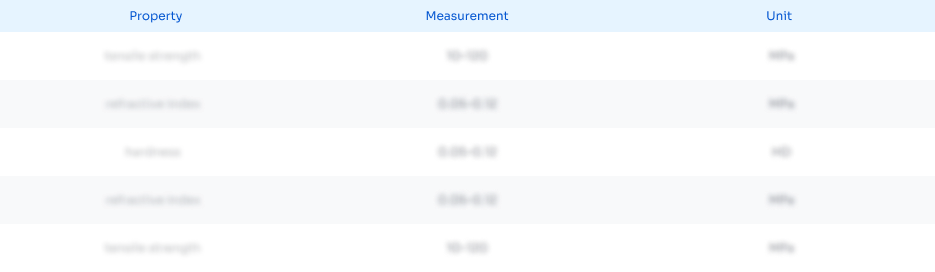
Abstract
Description
Claims
Application Information
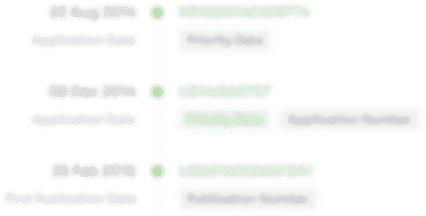
- R&D Engineer
- R&D Manager
- IP Professional
- Industry Leading Data Capabilities
- Powerful AI technology
- Patent DNA Extraction
Browse by: Latest US Patents, China's latest patents, Technical Efficacy Thesaurus, Application Domain, Technology Topic.
© 2024 PatSnap. All rights reserved.Legal|Privacy policy|Modern Slavery Act Transparency Statement|Sitemap