Human-face identifying method and device
A face recognition and face image technology, applied in the field of recognition, can solve the problems of large number of training samples, computational complexity and difficulty of similarity learning, etc., to improve accuracy, reduce computational complexity, and reduce data volume. Effect
- Summary
- Abstract
- Description
- Claims
- Application Information
AI Technical Summary
Problems solved by technology
Method used
Image
Examples
Embodiment 1
[0056] see figure 1 , which shows a flow chart of Embodiment 1 of a face recognition method provided by the present application, including:
[0057] Step S101: performing a first classification process on the face image samples to obtain a training sample group and a test sample group respectively;
[0058] In the process of using a class of support vector machines to recognize face images, the class of support vector machines is firstly trained.
[0059] The face image samples in the database are divided into two categories, including: training sample group and test sample group.
[0060] The training sample group is used to train a class of support vector machines to make its parameters more precise and more accurate. The test sample group is used to test the one-class support vector machine after the training, so as to detect the recognition accuracy of the one-class support vector machine after the training.
[0061] Step S102: Perform a second classification process on...
Embodiment 2
[0077] see figure 2 , showing a flow chart of Embodiment 2 of a face recognition method provided by the present application, figure 1 In the flow chart shown, step S102 includes:
[0078] Step S1021: Divide the training samples in the training sample group into at least two subsets according to preset classification conditions, and each subset corresponds to one type of data;
[0079] According to the classification condition, the classification condition may be various conditions related to face distinction such as age, gender, and race.
[0080] The training samples in the training sample group are divided into multiple subsets, and each subset is a class of data.
[0081] It is assumed that there is a set of face training samples, which are displayed in the form of data, {(x 1 ,v 1 ),…,(x i ,v i ),…,(x n ,v n )}, where x i ∈ R D , v i ∈{1,2,...,C}.
[0082] v i is x i The category label for the class indicating the category of the classification. In this emb...
Embodiment 3
[0096] see image 3, showing a flow chart of Embodiment 3 of a face recognition method provided by the present application, figure 2 In the flow chart shown, step S103 includes:
[0097] Step S1031: select the kernel function as the Gaussian radial basis function, and preset kernel parameter values;
[0098] The Gaussian radial basis function is selected as the kernel function of a class of support vector machine, and the Gaussian radial basis function k(z, z′)=e -σ||z-z′|| , where the σ is a kernel parameter, and the value of the kernel parameter is preset according to the actual situation.
[0099] Step S1032: input the training sample pair into the kernel function, train a type of support vector machine, and obtain model coefficients;
[0100] After specifying the experimental parameters, use the Gaussian radial basis function as the kernel function, input the training samples in the training sample pair group, train this type of execution vector machine, and obtain the...
PUM
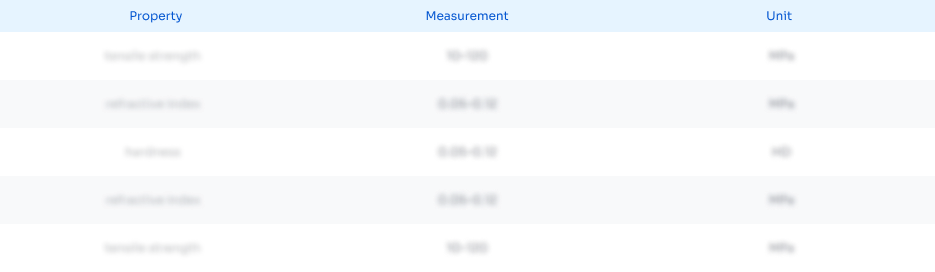
Abstract
Description
Claims
Application Information
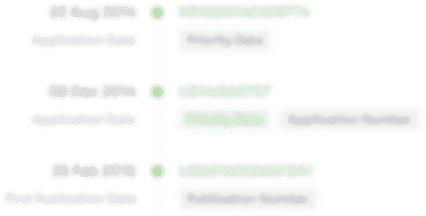
- R&D
- Intellectual Property
- Life Sciences
- Materials
- Tech Scout
- Unparalleled Data Quality
- Higher Quality Content
- 60% Fewer Hallucinations
Browse by: Latest US Patents, China's latest patents, Technical Efficacy Thesaurus, Application Domain, Technology Topic, Popular Technical Reports.
© 2025 PatSnap. All rights reserved.Legal|Privacy policy|Modern Slavery Act Transparency Statement|Sitemap|About US| Contact US: help@patsnap.com