Power quality disturbance recognition and classification method based on PSO (Particle Swarm Optimization) for SVM (Support Vector Machine)
A technology of power quality disturbance and classification method, applied in the direction of measuring electrical variables, character and pattern recognition, measuring electricity, etc., can solve the problems of not finding parameters, searching for optimization, etc., to achieve high accuracy, accurate and reliable identification, and improve training speed. The effect of classification accuracy
- Summary
- Abstract
- Description
- Claims
- Application Information
AI Technical Summary
Problems solved by technology
Method used
Image
Examples
Embodiment Construction
[0022] Below in conjunction with accompanying drawing and specific embodiment the present invention is described in further detail:
[0023] Such as Figures 1 to 7 As shown, the power quality disturbance identification and classification method based on PSO optimized SVM includes the following steps:
[0024] a. Establish a signal model containing common dynamic disturbance signals. Common disturbance signals include five types of voltage swell signals, voltage sag signals, temporary voltage interruption signals, transient pulse signals and transient oscillation signals. Table 1 shows the five A model of a common dynamic disturbance signal and the corresponding parameter settings.
[0025] Table 1 Five common dynamic disturbance signal models
[0026]
[0027] The disturbance signal is extracted from the input voltage signal by complex wavelet transform, and the disturbance signal is decomposed by multi-scale complex wavelet by constructing the Db4 orthogonal compact sup...
PUM
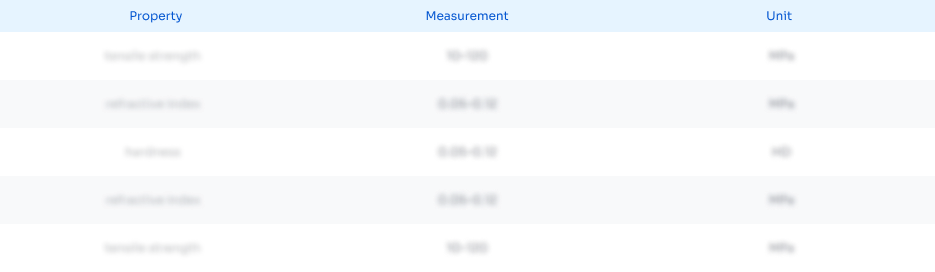
Abstract
Description
Claims
Application Information
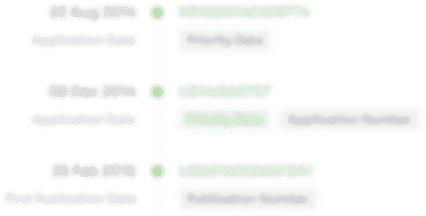
- R&D Engineer
- R&D Manager
- IP Professional
- Industry Leading Data Capabilities
- Powerful AI technology
- Patent DNA Extraction
Browse by: Latest US Patents, China's latest patents, Technical Efficacy Thesaurus, Application Domain, Technology Topic, Popular Technical Reports.
© 2024 PatSnap. All rights reserved.Legal|Privacy policy|Modern Slavery Act Transparency Statement|Sitemap|About US| Contact US: help@patsnap.com