Method for reconstructing non-convex compression congnitive image based on evolution multi-target optimization
A multi-objective optimization and compressed sensing technology, applied in the field of non-convex compressed sensing image reconstruction, it can solve the problems of weak sparseness, violation of the theoretical basis of compressed sensing, and poor image quality.
- Summary
- Abstract
- Description
- Claims
- Application Information
AI Technical Summary
Problems solved by technology
Method used
Image
Examples
Embodiment Construction
[0087] Such as figure 1 As shown, the non-convex compressive sensing image reconstruction method based on evolutionary multi-objective optimization includes at least the following steps:
[0088] Step 101: Obtain the total observation vector.
[0089] Such as figure 2 As shown, the specific implementation of this step is as follows:
[0090] 1.1) Input test image, carry out wavelet transform to test image;
[0091] 1.2) Keep the low-frequency wavelet coefficient C l , the high-frequency wavelet coefficients are divided into blocks for every 8 pixels to obtain the block signal:
[0092] 1.2.1) Divide the high-frequency wavelet coefficients into 8×8 blocks;
[0093] 1.2.2) Take one element of each 8×8 block in the order from left to right and from top to bottom (also in the order from left to right and top to bottom) to form a broken block signal, In this way, multiple scattered block signals can be obtained;
[0094] 1.3) Use the orthogonal random Gaussian observation m...
PUM
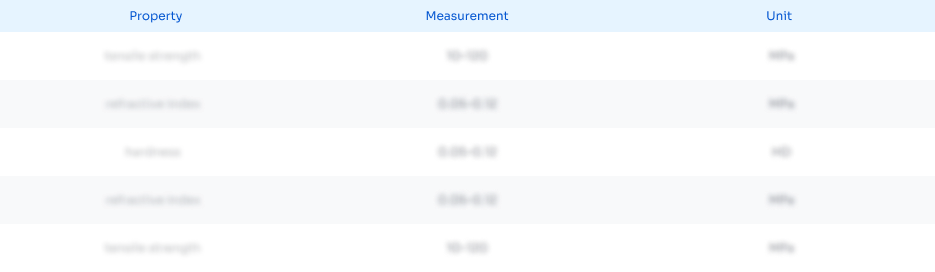
Abstract
Description
Claims
Application Information
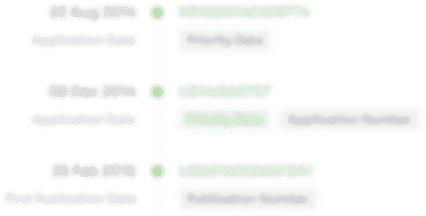
- R&D Engineer
- R&D Manager
- IP Professional
- Industry Leading Data Capabilities
- Powerful AI technology
- Patent DNA Extraction
Browse by: Latest US Patents, China's latest patents, Technical Efficacy Thesaurus, Application Domain, Technology Topic.
© 2024 PatSnap. All rights reserved.Legal|Privacy policy|Modern Slavery Act Transparency Statement|Sitemap