Machine fault prediction method based on MFCC feature extraction
A technology for machine failure and prediction methods, which is applied in instruments, special data processing applications, electrical digital data processing, etc., and can solve problems such as the randomness, time variability, nonlinearity, large error, and slow convergence speed of machine failure characteristics.
- Summary
- Abstract
- Description
- Claims
- Application Information
AI Technical Summary
Problems solved by technology
Method used
Image
Examples
Embodiment 1
[0057] Embodiment 1: In this fault prediction method, the sound signal characteristics of the current machine operation are obtained through the acoustic sensor installed on the machine. After the sound signal is preprocessed, it is subjected to Mel transformation to obtain its MFCC feature vector. Predict the health status of the machine according to the obtained MFCC feature vector. The specific clustering process is that the support vector machine clusters the MFCC features extracted when the machine is running and the sample data stored in the normal operation of the machine before, and the clustering results are analyzed by the voting method. analysis to predict machine failures.
[0058] Said Mel is the frequency cepstral coefficient, expressed as Mel Frequency Cestrum Coefficient in English, and MFCC in English. The English name of the support vector machine is support vector machine, and the English abbreviation is SVM.
[0059] Such as figure 1 Shown, the present in...
PUM
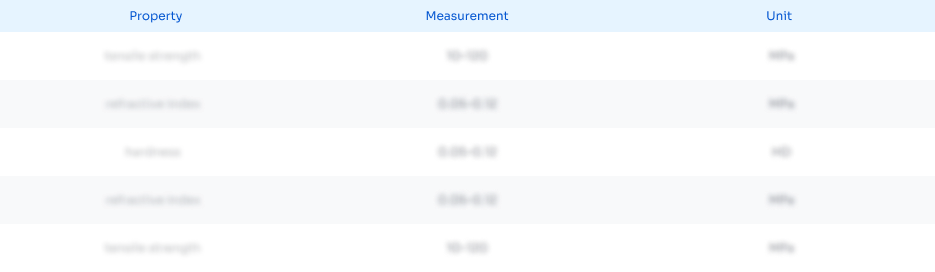
Abstract
Description
Claims
Application Information
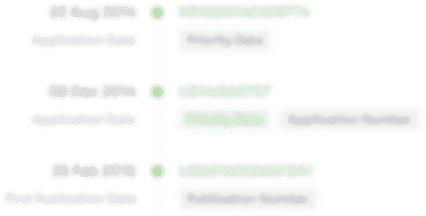
- R&D
- Intellectual Property
- Life Sciences
- Materials
- Tech Scout
- Unparalleled Data Quality
- Higher Quality Content
- 60% Fewer Hallucinations
Browse by: Latest US Patents, China's latest patents, Technical Efficacy Thesaurus, Application Domain, Technology Topic, Popular Technical Reports.
© 2025 PatSnap. All rights reserved.Legal|Privacy policy|Modern Slavery Act Transparency Statement|Sitemap|About US| Contact US: help@patsnap.com