A Spectrum Detection Method Based on Compressive Sensing under Blind Sparse Condition
A compressed sensing and spectrum detection technology, applied in electrical components, transmission monitoring, transmission systems, etc., can solve the problems of slow detection algorithm convergence speed, increased algorithm complexity, missed spectrum detection, etc., to achieve convergence, real-time The effect of good performance and low complexity
- Summary
- Abstract
- Description
- Claims
- Application Information
AI Technical Summary
Problems solved by technology
Method used
Image
Examples
specific Embodiment approach 1
[0029] Specific implementation mode 1: A spectrum detection method for compressive sensing under blind sparse conditions in this implementation mode is specifically prepared according to the following steps:
[0030] Step 1. According to the compressive sensing theory, a mathematical model is established for the antenna receiving signal x with a length of N Carry out optimization iterative solution; Wherein, measurement matrix is Θ, and the signal after compressed sampling is y, and f is the sparse base coefficient sought, Ψ is transformation base matrix, and Φ is Gaussian random matrix;
[0031] Step 2, under the premise that the measurement matrix is Θ satisfying the RIP property of the matrix, the mathematical model obtained in step 1 is simplified to obtain the convex optimization problem of the signal;
[0032] Step 3: Use the greedy pursuit algorithm to detect the correlation of the convex optimization problem of the signal, and obtain an element with the greatest c...
specific Embodiment approach 2
[0042] Specific embodiment 2: The difference between this embodiment and specific embodiment 1 is: in step 1, according to the compressed sensing theory, the mathematical model established by the antenna receiving signal x with a length of N The specific problem for optimization iterative solution is described as follows:
[0043] (1) The length of the signal received by the antenna is N, and the compressed sampling rate is Randomly select K sub-frequency bands from N frequency bands as frequency bands occupied by authorized users, and M is the number of compressed sampling points;
[0044] (2) The N×1-dimensional noise-free signal x_o obtained by the inverse Fourier transform of the frequency band occupied by authorized users; x_o∈R N , the method of inverse Fourier transform is x_o=Ψf, f is the sparse basis coefficient sought;
[0045] (3) If there are only K elements in f that are non-zero, the signal x_o is said to be sparse under the transformation basis of Ψ, and the...
specific Embodiment approach 3
[0053] Specific embodiment three: the difference between this embodiment and specific embodiment one or two is that in step two, under the premise that the measurement matrix is Θ satisfying the RIP property of the matrix, the mathematical model obtained in step one is simplified to obtain the convex optimization problem of the signal The specific process is:
[0054] Although the 0-norm algorithm of compressed sensing is optimal, it is an NP-hard problem. In order to find the sparsest solution, it is necessary to exhaustively This is a possibility, and the complexity of the algorithm is extremely high; relevant data show that under the premise that the measurement matrix is Θ and satisfies the RIP property of the matrix, use MATLAB software as the simulation software, input: sampling vector y, measurement matrix Θ=ΦΨ of compressed sensing, iteration The termination threshold s, the purpose of the algorithm in the invention is to output the spectrum sensing result under t...
PUM
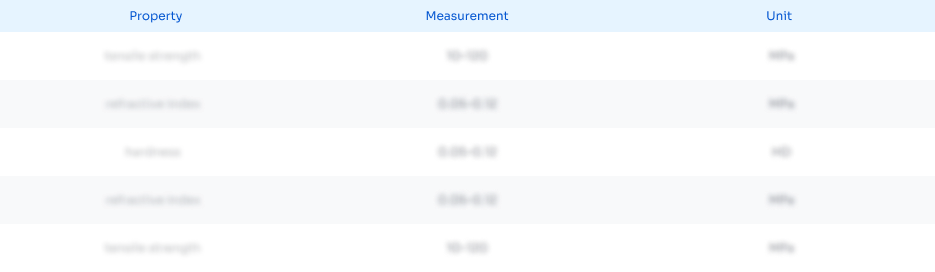
Abstract
Description
Claims
Application Information
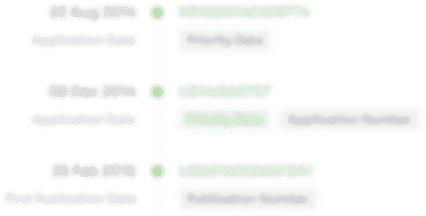
- R&D Engineer
- R&D Manager
- IP Professional
- Industry Leading Data Capabilities
- Powerful AI technology
- Patent DNA Extraction
Browse by: Latest US Patents, China's latest patents, Technical Efficacy Thesaurus, Application Domain, Technology Topic.
© 2024 PatSnap. All rights reserved.Legal|Privacy policy|Modern Slavery Act Transparency Statement|Sitemap