Brain MR Image Segmentation Method Combining Weighted Neighborhood Information and Offset Field Restoration
A technology of image segmentation and neighborhood information, applied in image analysis, image data processing, instruments, etc., can solve problems such as high computational complexity, easy misclassification of segmentation boundaries or slender topological structures, and failure to consider image structure information, etc. , to reduce the influence of noise and improve the robustness
- Summary
- Abstract
- Description
- Claims
- Application Information
AI Technical Summary
Problems solved by technology
Method used
Image
Examples
Embodiment 1
[0073] figure 2 is a comparison graph of the segmentation results of the synthetic image of the brain. figure 2 .a is the original image, the image noise level is 3%, and the INU level is 0%. From the figure, we can find that it contains strong noise. Respectively figure 2 .a using FCM algorithm, CLIC algorithm and the method provided by the present invention to segment the image. figure 2 .b is the segmentation result of the FCM algorithm. It can be seen that the FCM algorithm is sensitive to noise because it only considers the grayscale information of a single image pixel. figure 2 .c is the segmentation result of the CLIC algorithm. This algorithm only uses Gaussian filtering to reduce the influence of noise, and the Gaussian kernel is isotropic, which is powerless to maintain large noise and slender topological structure, resulting in partial gray matter Wrongly classified as white matter misclassification. figure 2 .d is the result obtained by the method of the ...
Embodiment 2
[0075] image 3 It is a comparison of segmentation results of synthetic images of the brain. image 3 .a is the original image with a noise level of 5% and an INU level of 0%, with stronger noise in the figure. Respectively image 3 .a using FCM algorithm, CLIC algorithm and the method provided by the present invention to segment the image. image 3 .b is the segmentation result of the FCM algorithm, image 3 .c is the segmentation result of the CLIC algorithm. It can be seen that the algorithm segmentation fails due to the influence of strong noise. image 3 .d is the result that the inventive method obtains, image 3 .e is the standard segmentation result. It can be seen that the anisotropic neighborhood information can have better robustness to noise, thus obtaining a more ideal segmentation result.
Embodiment 3
[0077] Figure 4 It is a comparison of segmentation results of synthetic images of the brain. Figure 4 .a is the original image with a noise level of 5% and an INU level of 80%. The image not only contains strong noise but also has strong gray scale inhomogeneity. Respectively Figure 4 .a using FCM algorithm, CLIC algorithm and the method provided by the present invention to segment the image. Figure 4 .b is the segmentation result of the FCM algorithm, and the segmentation fails due to the influence of the offset field. Figure 4 .c is the segmentation result of the CLIC algorithm. This method uses small neighborhood information to reduce the influence of the offset field, but it is still affected by noise, resulting in poor accuracy of the algorithm. Figure 4 .d is the segmentation result of the method of the present invention, Figure 4 .e is the result of the standard segmentation. Since the present invention couples the offset field information, the uneven gray fi...
PUM
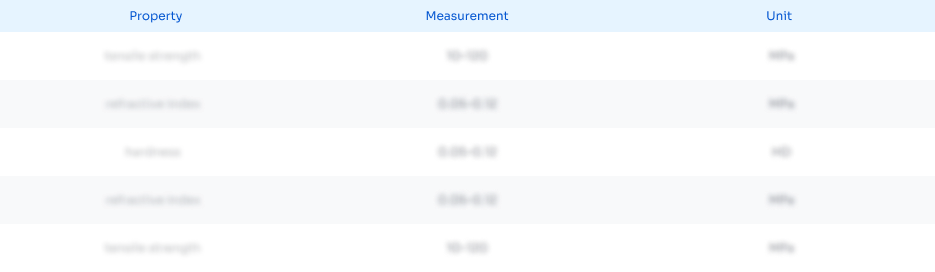
Abstract
Description
Claims
Application Information
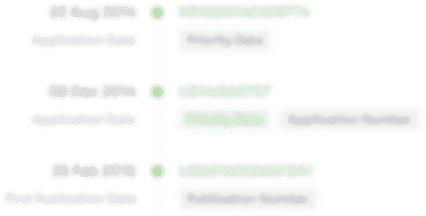
- R&D Engineer
- R&D Manager
- IP Professional
- Industry Leading Data Capabilities
- Powerful AI technology
- Patent DNA Extraction
Browse by: Latest US Patents, China's latest patents, Technical Efficacy Thesaurus, Application Domain, Technology Topic, Popular Technical Reports.
© 2024 PatSnap. All rights reserved.Legal|Privacy policy|Modern Slavery Act Transparency Statement|Sitemap|About US| Contact US: help@patsnap.com