Lithium ion battery remaining service life prediction method based on AR ensemble learning model
A lithium-ion battery and life prediction technology, applied in the direction of measuring electricity, measuring devices, measuring electrical variables, etc., can solve problems such as low stability and limited accuracy
- Summary
- Abstract
- Description
- Claims
- Application Information
AI Technical Summary
Problems solved by technology
Method used
Image
Examples
specific Embodiment approach 1
[0049] Specific implementation mode one: combine figure 1 , the process of the lithium-ion battery remaining life prediction method based on the AR integrated learning model is:
[0050] Step 1: According to the capacity failure threshold of the battery, the end of life time of the battery is obtained from the capacity data of the battery, and the time quantification is represented as the number of cycles of battery charge and discharge; a% of the number of cycles at the end of life is used as the starting point for prediction; Extract capacity data, use it as the original input data F for order judgment, and standardize F to obtain standardized data Y;
[0051] Zero-meanization: calculate the mean value Fmean of the input data F, and obtain the zero-meanization sequence f=F-Fmean;
[0052] Variance standardization: Find the standard deviation σ of the sequence f f , get standardized data Y=f / σ f ;
[0053] Step 2: Calculate the 0-step autocovariance of the standardized da...
specific Embodiment approach 2
[0086] Specific implementation mode two: the specific operation steps of step 4 of this embodiment mode are:
[0087] Construct the matrix of the Yule-Wallker equation in the following form:
[0088] ρ 1 ρ 2 · · · ρ p = 1 ρ 1 · · · ρ p - 1 ...
specific Embodiment approach 3
[0092] Specific implementation mode three: the specific operation steps of step 5 of the present implementation mode are:
[0093] Step 5.1 is calculated by autocorrelation coefficient:
[0094] S=[R 0 ,R(1),R(2),R(3)] (9)
[0095] S is a vector composed of 0-3 step autocorrelation coefficients, R 0 , R(1), R(2), R(3) are 0-3 step autocorrelation coefficients respectively;
[0096] Step 5.2 calculates the Toeplitz matrix Toeplitz matrix according to S:
[0097] G=toeplitz(S) (10)
[0098] Among them, G is the Toeplitz matrix of vector S;
[0099] Step 5.3 calculates the intermediate vector W:
[0100] W=G -1 ·[R(1),R(2),R(3),R(4)] T (11)
[0101] Compute the model residual variance:
[0102] σ p 2 = 1 L 1 - p Σ t = p + ...
PUM
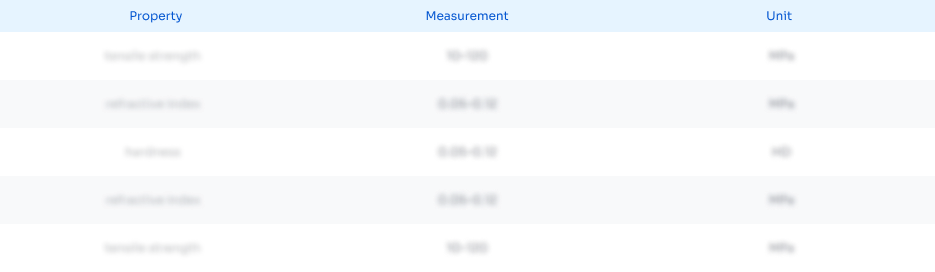
Abstract
Description
Claims
Application Information
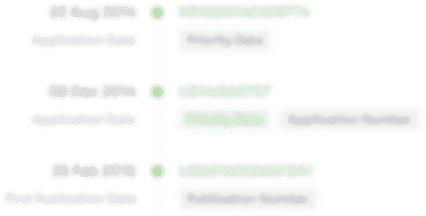
- R&D
- Intellectual Property
- Life Sciences
- Materials
- Tech Scout
- Unparalleled Data Quality
- Higher Quality Content
- 60% Fewer Hallucinations
Browse by: Latest US Patents, China's latest patents, Technical Efficacy Thesaurus, Application Domain, Technology Topic, Popular Technical Reports.
© 2025 PatSnap. All rights reserved.Legal|Privacy policy|Modern Slavery Act Transparency Statement|Sitemap|About US| Contact US: help@patsnap.com