Support vector machine kernel function selection method under sparse representation and application thereof
A support vector machine and sparse representation technology, applied in complex mathematical operations, computer components, instruments, etc., to achieve the effect of strong generalization ability
- Summary
- Abstract
- Description
- Claims
- Application Information
AI Technical Summary
Problems solved by technology
Method used
Image
Examples
Embodiment 1
[0037] Embodiment 1: Sewage detection experimental data
[0038] Step A: 57 sets of sample data were collected in a sewage treatment plant and preprocessed to make the norm of the sample data less than 1; then DO (dissolved oxygen), BOD5, membrane pressure difference, and water production in the sewage were selected The seven parameters of daily treatment capacity, actual residence time, and influent load are used as input variables, and COD and NH4+-N indicators in the effluent treated by membrane biotechnology are used as output variables;
[0039] Step B: select and construct different kernel functions that meet the Mercer conditions, and establish a complete sparse dictionary of SVM kernel functions;
[0040] Step C: select 42 sets of sample data as training samples, 15 sets of sample data as test samples, and then use the sparse representation theory to obtain the sparse coding of the sample data under the sparse dictionary;
[0041] Step D: select the SVM kernel functio...
Embodiment 2
[0047] Embodiment 2: Servo Data Set (servo data set)
[0048] Step A: This data set comes from the UCI database, with a total of 167 sets of data, and it is preprocessed to make the norm of the sample data less than 1; then select 4 motor types, screw specifications, amplification factor p, and amplification factor v is the input variable, and the servo motor type is the output variable;
[0049] Step B: select and construct different kernel functions that meet the Mercer conditions, and establish a complete sparse dictionary of SVM kernel functions;
[0050] Step C: select 132 sets of sample data as training samples, 35 sets of sample data as test samples, and then use the sparse representation theory to obtain the sparse coding of the sample data under the sparse dictionary;
[0051] Step D: select SVM kernel function type number n=1 through sparse coding, SVM kernel function type RBF radial basis kernel function k RBF , at this time ρ=1;
[0052] E step: utilize particle s...
Embodiment 3
[0054] Embodiment three: Yacht Hydrodynamics Data Set (yacht hydrodynamics data set)
[0055] Step A: This data set comes from the UCI database, with a total of 308 sets of data, which are used to predict the hydrodynamic performance of the yacht, the size and speed of the sailing yacht; preprocess it so that the norm of the sample data is less than 1; then select the center of buoyancy Six input variables are longitudinal position, rhombus coefficient, ship length displacement ratio, ship width displacement ratio, ship length width ratio, and Froude coefficient, and the displacement per unit weight of residual resistance is output variable;
[0056] Step B: select and construct different kernel functions that meet the Mercer conditions, and establish a complete sparse dictionary of SVM kernel functions;
[0057] Step C: Select 240 sets of sample data as training samples, 68 sets of sample data as test samples, and then use the sparse representation theory to obtain the sparse...
PUM
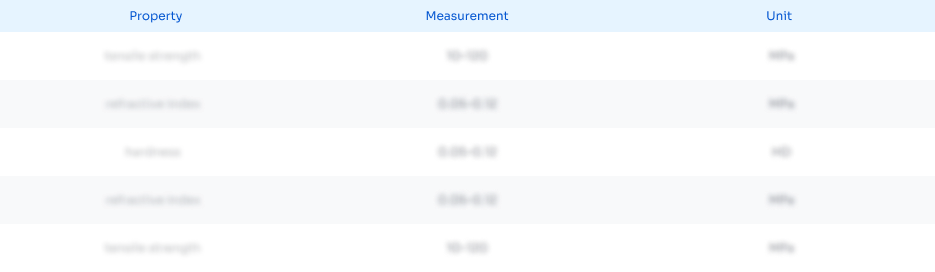
Abstract
Description
Claims
Application Information
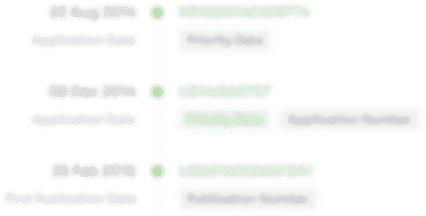
- R&D Engineer
- R&D Manager
- IP Professional
- Industry Leading Data Capabilities
- Powerful AI technology
- Patent DNA Extraction
Browse by: Latest US Patents, China's latest patents, Technical Efficacy Thesaurus, Application Domain, Technology Topic.
© 2024 PatSnap. All rights reserved.Legal|Privacy policy|Modern Slavery Act Transparency Statement|Sitemap