An Adaptive Kernel Clustering Image Segmentation Method
An image segmentation and kernel clustering technology, applied in the field of image processing, can solve problems such as wrong segmentation results and inaccurate cluster numbers, and achieve the effects of reducing time consumption, reducing the possibility of local convergence, and reducing sensitivity
- Summary
- Abstract
- Description
- Claims
- Application Information
AI Technical Summary
Problems solved by technology
Method used
Image
Examples
Embodiment Construction
[0040] The present invention will be further described below in conjunction with the accompanying drawings.
[0041] Such as figure 1 As shown, an adaptive kernel clustering image segmentation method, for an image sample gray value set containing n elements X={x 1 ,x 2 ,...,x n},, each element in the sample has s characteristic attributes, then each sample element can use the vector x k =[x k1 ,x k2 ,...,x ks ] T ∈R s Represents, where T represents the matrix transpose, and the vector x k The value of each dimension of is the value of its corresponding characteristic attribute. Now divide the sample set X into c clusters, where the value range of c is 1k The membership degrees of c classifications are expressed as vectors, then the membership of n elements to c classifications can be divided by matrix U=[u ik ] c×n Indicates that U ∈ R cn . at the same time u ik Constraints are met:
[0042]
[0043] use theta i Indicates the cluster center, and all cluster ...
PUM
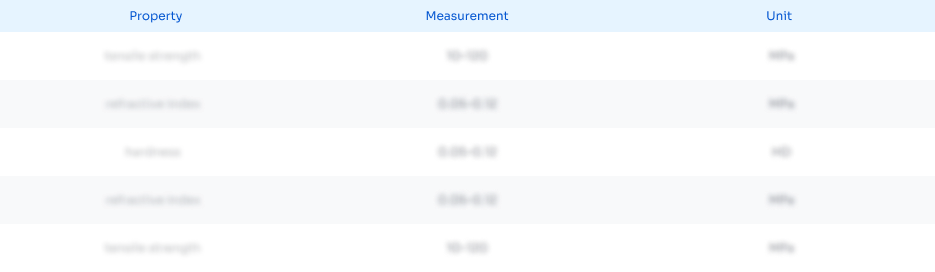
Abstract
Description
Claims
Application Information
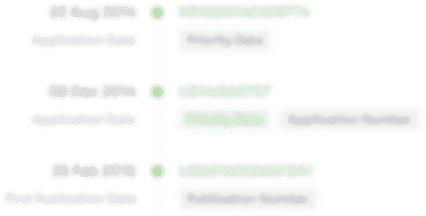
- R&D
- Intellectual Property
- Life Sciences
- Materials
- Tech Scout
- Unparalleled Data Quality
- Higher Quality Content
- 60% Fewer Hallucinations
Browse by: Latest US Patents, China's latest patents, Technical Efficacy Thesaurus, Application Domain, Technology Topic, Popular Technical Reports.
© 2025 PatSnap. All rights reserved.Legal|Privacy policy|Modern Slavery Act Transparency Statement|Sitemap|About US| Contact US: help@patsnap.com